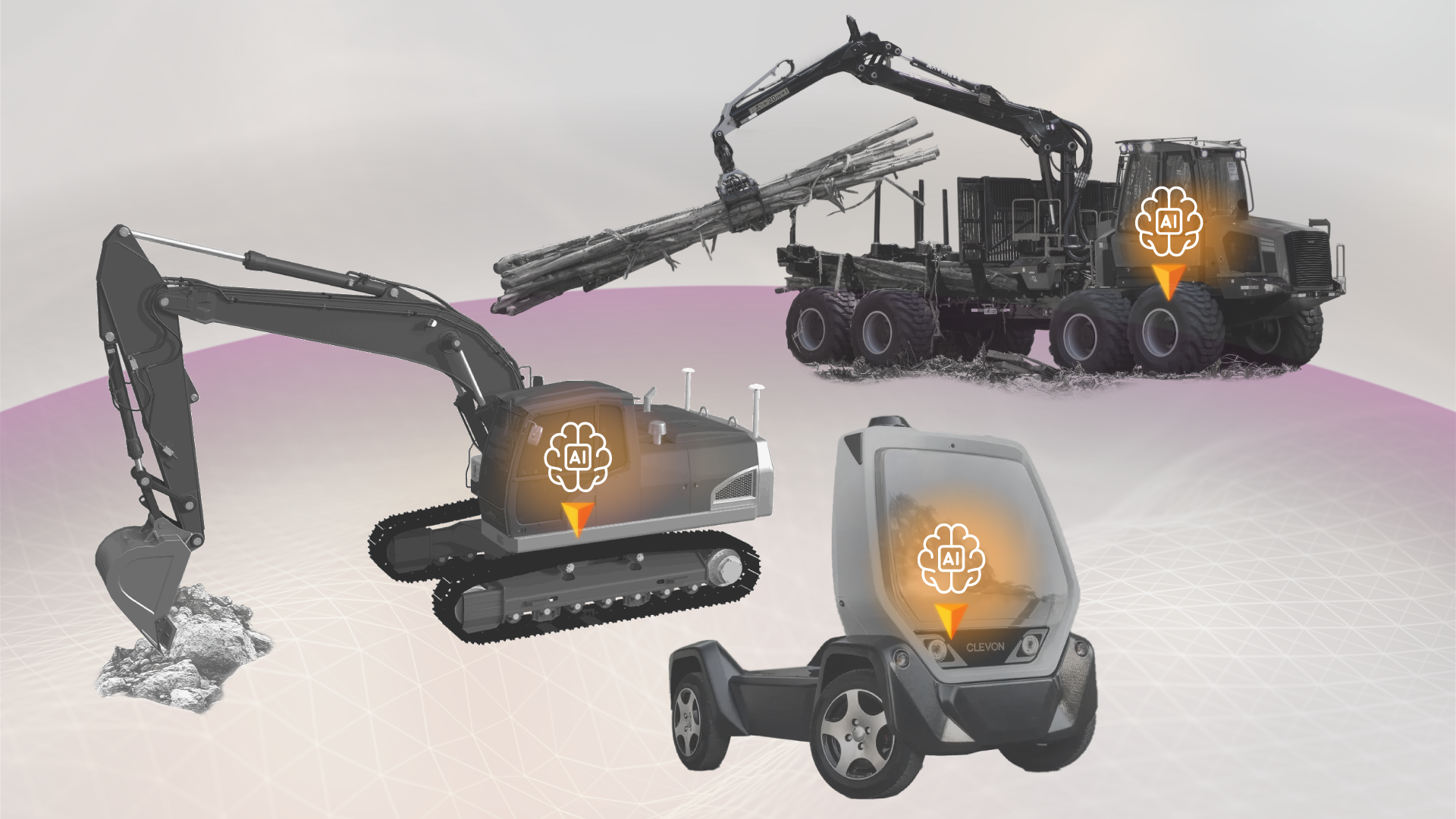
Algoryx is envisioning a plan for bringing full autonomy to heavy off-road machines, even in the most complex and unpredictable environments. At the heart of this visionary approach is the idea that a safe and efficient AI-powered control system needs to operate in two worlds: the real one and a physics-based simulation running in parallel.
The heavy off-road machines of the future will undoubtedly be self-operating. But how, and when, will this future arrive?
Two broad industry trends are emerging: heavy machines are becoming smarter and robots are becoming more mobile. Gradually, the two domains are converging. When an excavator is enhanced with an AI-based control system, it effectively becomes a type of autonomous mobile robot.
AI that safely handles the unexpected
However, existing robotic control technology isn’t advanced enough to tackle unstructured terrain. For example, a self-driving excavator must adjust its scooping actions depending on whether it encounters loose soil or rocks of various shapes and sizes. The machine, drawing on sensor data, needs to make quick judgments about its environment and adapt its actions in real time. This kind of continuous adaptation requires a level of environmental understanding that traditional automated robotics simply can’t achieve.
Researchers have explored deep neural networks and other self-learning AI methods to implement autonomous control of heavy machinery with promising results. However, fundamental challenges remain before the technology is ready for large-scale industrial applications.
“Today, physics-based simulation is used to test and train control systems and advanced AI models, so-called deep neural networks. The use of simulation is a safe and efficient way to cover a wide range of scenarios, but it remains difficult to ensure safe behavior in situations that differ significantly from the training cases,” says Martin Servin, senior principal scientist at Algoryx, and associate professor in physics at Umeå University.
The critical questions remain: How can AI effectively translate sensor data into a clear understanding of a machine’s surroundings? And once it has that understanding, how can it plan and execute the machine’s movements efficiently and safely?
Patterns and principles – how will AI understand the world?
Some practitioners believe that a sufficiently powerful AI system will eventually develop a working understanding of how real-world objects interact dynamically purely by being trained on various types of sensor data.
Others take a different view. These experts argue that AI designed to control robotics in complex environments must have a deep, built-in understanding of the laws of physics. With this understanding, the system can model the machine and its environment mathematically, allowing it to predict movements and forces with speed, precision, and robustness.
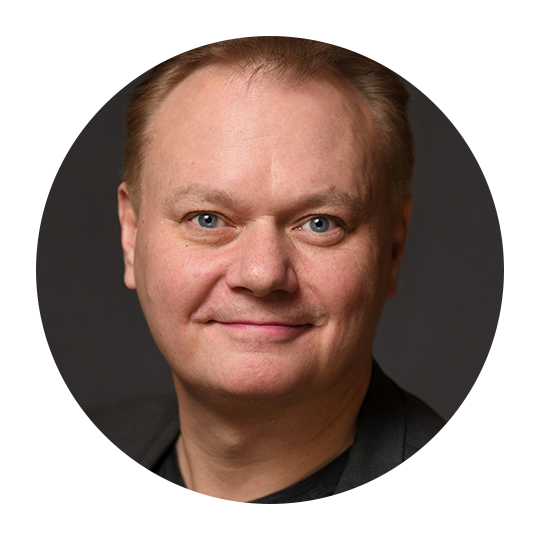
“Now, we are developing machines that comprehend the world through fundamental physical principles.”
Kenneth Bodin, CEO and co-founder, Algoryx
Algoryx, a pioneer in real-time physics simulation, is firmly positioned in the latter camp. Many of the world’s leading machine manufacturers and researchers rely on Algoryx’s high-fidelity physics simulation software, AGX Dynamics, as a virtual training ground for autonomous control systems. But for Algoryx, digital physics isn’t just a tool for AI training—it should be embedded directly into the working machine’s autonomy systems.
“Machines are designed to extend human capabilities across large scales of time, speed, range, and force. Now, we are developing machines that comprehend the world through fundamental physical principles. This integration will enable autonomous systems to anticipate outcomes and make informed decisions, even amidst the complexity of interacting scales — something that traditional neural networks cannot achieve alone,” says Algoryx CEO Kenneth Bodin.
Tackling perception-to-action challenges in €8 Million project
To move closer to realizing this vision, Algoryx has joined an elite group of European industry partners and academic researchers in the €8 million EU-funded XSCAVE project. The industry partners include Swedish forestry machine manufacturer Komatsu Forest, Finnish earthmoving automation company Novatron, and Estonian mobile robotics firm Clevon.
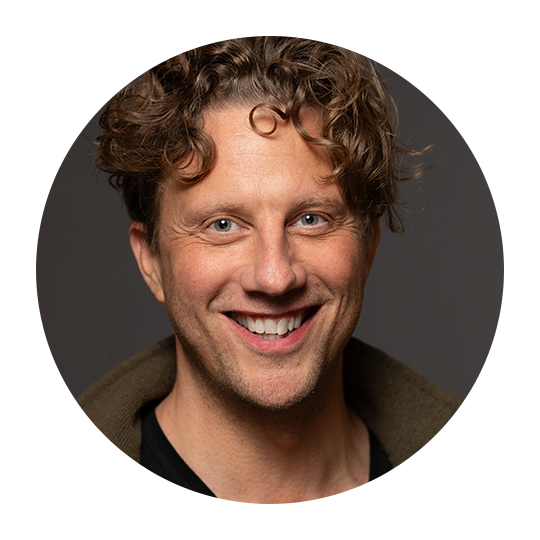
“When we embed computational models and equation solvers for the physics, we make the AI informed about cause and effect”
Martin Servin, senior principal scientist and co-founder, Algoryx
XSCAVE, short for “explainable, safe, contact-aware planning and control for heavy machinery manipulation and navigation,” relies on Algoryx’s digital physics as a key component of its perception-to-action technology. First, Algoryx will create physics-based simulators used to train AI systems. Later in the project, we will develop a lightweight version of the physics simulation engine, designed to be embedded directly into the machines to provide real-time feedback during operation.
“When we embed computational models and equation solvers for the physics, we make the AI informed about cause and effect, and a tool for predicting the probable outcome of planned movements before they are executed. This makes it possible to rule out options associated with an unacceptable risk of damage or negative environmental impact. At the same time, we believe this is a way to achieve higher precision and efficiency,” says Martin Servin.
The goal of the project is to create autonomous terrain machines with unprecedented abilities to plan, control, and adapt their actions based on the task at hand and the terrain they’re navigating. In the final stages of XSCAVE, the new control system technology will be implemented in machines like a forestry forwarder and an excavator, demonstrating the groundbreaking capabilities of this AI-driven system.
The academic partners involved in XSCAVE bring world-leading expertise in autonomous control systems, machine learning, physics simulation, and computer vision. The University of Tartu (Estonia) coordinates the project, with contributions from Tampere University (Finland), Aalto University (Finland), Umeå University (Sweden), FZI Forschungszentrum Informatik (Germany), and Czech Technical University in Prague (Czechia).
For the XSCAVE project partners, a hybrid approach between neural network pipelines and physics-derived priors is the most promising way to build autonomous systems that can handle the complex work tasks of construction, forestry, and off-road transportation. It is also the best way to build autonomous systems that will be safe and explainable.
- Algoryx wins Ignite Hero of the Year at Ignite Awards 2024Ignite Sweden. a national non-profit organization for partnerships between startups, large companies, and the public sector, has awarded Algoryx the title of Ignite Hero of the Year.
- Heavy offroad autonomy with embedded physicsPursuing our technology vision with industry and academic partners
- Robots with a Sense for Physics: Algoryx’s Vision for Heavy Offroad Autonomy, and a Plan to Achieve ItAlgoryx is envisioning a plan for bringing full autonomy to heavy off-road machines, even in the most complex and unpredictable […]