2025
|
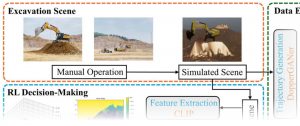 | Zhao, Qianyou; Gao, Le; Wu, Duidi; Lei, Yihao; Wang, Lingyu; Qi, Jin; Hu, Jie: E-GCDT: advanced reinforcement learning with GAN-enhanced data for continuous excavation system. In: Applied Intelligence, vol. 55, no. 413, 2025. @article{nokey,
title = {E-GCDT: advanced reinforcement learning with GAN-enhanced data for continuous excavation system},
author = {Qianyou Zhao and Le Gao and Duidi Wu and Yihao Lei and Lingyu Wang and Jin Qi and Jie Hu},
url = {https://rdcu.be/d9k0a},
doi = {https://doi.org/10.1007/s10489-025-06308-5},
year = {2025},
date = {2025-01-28},
urldate = {2025-01-28},
journal = {Applied Intelligence},
volume = {55},
number = {413},
abstract = {The automation of excavator operations entails the development and implementation of systems that allow excavators to execute tasks autonomously, thereby significantly reducing the need for human intervention. By integrating advanced sensors and artificial intelligence algorithms, these systems aim to increase operational efficiency, safety, and precision in construction and mining. However, previously developed methods have two weaknesses. First, existing automated excavator systems struggle with adapting to diverse and complex environmental conditions and with precision in control mechanisms. Second, operating an excavator involves multiple, repeated decisions that need to be modeled, planned, and executed in real time. However, there is a significant lack of comprehensive datasets that reflect real-world excavation operations to support this process. In this paper, we present an innovative system named E-GCDT. This system integrates the DoppelGANger module, which generates action time series by emulating human-mined trajectories through a generative adversarial mechanism and replays them in a simulation environment, ultimately expanding the dataset to 155 continuous mining trajectories. Furthermore, E-GCDT integrates terrain features into the decision-making process with the contrastive language-image pre-training model (CLIP), in which the decision transformer optimizes trajectory planning for efficient and accurate continuous excavation tasks. E-GCDT uniquely integrates advanced data augmentation and terrain awareness, developing an advanced Markov decision framework (DT) for continuous excavation tasks. The experimental results of a bulldozer verify that the efficiency of E-GCDT surpasses human efficiency. This system sets a new standard for continuous autonomous mining, and this study provides a new perspective on the application of reinforcement learning in industrial environments.},
keywords = {},
pubstate = {published},
tppubtype = {article}
}
The automation of excavator operations entails the development and implementation of systems that allow excavators to execute tasks autonomously, thereby significantly reducing the need for human intervention. By integrating advanced sensors and artificial intelligence algorithms, these systems aim to increase operational efficiency, safety, and precision in construction and mining. However, previously developed methods have two weaknesses. First, existing automated excavator systems struggle with adapting to diverse and complex environmental conditions and with precision in control mechanisms. Second, operating an excavator involves multiple, repeated decisions that need to be modeled, planned, and executed in real time. However, there is a significant lack of comprehensive datasets that reflect real-world excavation operations to support this process. In this paper, we present an innovative system named E-GCDT. This system integrates the DoppelGANger module, which generates action time series by emulating human-mined trajectories through a generative adversarial mechanism and replays them in a simulation environment, ultimately expanding the dataset to 155 continuous mining trajectories. Furthermore, E-GCDT integrates terrain features into the decision-making process with the contrastive language-image pre-training model (CLIP), in which the decision transformer optimizes trajectory planning for efficient and accurate continuous excavation tasks. E-GCDT uniquely integrates advanced data augmentation and terrain awareness, developing an advanced Markov decision framework (DT) for continuous excavation tasks. The experimental results of a bulldozer verify that the efficiency of E-GCDT surpasses human efficiency. This system sets a new standard for continuous autonomous mining, and this study provides a new perspective on the application of reinforcement learning in industrial environments. |
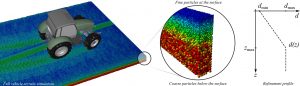 | Pogilus, Markus; Servin, Martin: Local particle refinement in terramechanical simulations. In: arxiv:2501.05300, 2025. @article{nokey,
title = {Local particle refinement in terramechanical simulations},
author = {Markus Pogilus and Martin Servin},
url = {https://arxiv.org/abs/2501.05300
https://arxiv.org/pdf/2501.05300},
year = {2025},
date = {2025-01-10},
urldate = {2025-01-10},
journal = {arxiv:2501.05300},
abstract = {The discrete element method (DEM) is a powerful tool for simulating granular soils, but its high computational demand often results in extended simulation times. While the effect of particle size has been extensively studied, the potential benefits of spatially scaling particle sizes are less explored. In this study, we systematically investigate a local particle refinement method's impact on reducing computational effort while maintaining accuracy. We first conduct triaxial tests to verify that bulk mechanical properties are preserved under local particle refinement. Then, we perform pressure-sinkage and shear-displacement tests, comparing our method to control simulations with homogeneous particle size. We evaluate 36 different DEM beds with varying aggressiveness in particle refinement. Our results show this approach, depending on refinement aggressiveness, can significantly reduce particle count by 2.3 to 25 times and simulation times by 3.1 to 43 times, with normalized errors ranging from 3.4% to 11%. The approach can keep a high resolution at the soil surface, where it is critical for accurately capturing the interaction while allowing larger particles below the surface. The results demonstrate that substantial computational savings can be achieved without significantly compromising simulation accuracy. This method can enhance the feasibility and efficiency of DEM simulations in terramechanics applications.},
keywords = {},
pubstate = {published},
tppubtype = {article}
}
The discrete element method (DEM) is a powerful tool for simulating granular soils, but its high computational demand often results in extended simulation times. While the effect of particle size has been extensively studied, the potential benefits of spatially scaling particle sizes are less explored. In this study, we systematically investigate a local particle refinement method's impact on reducing computational effort while maintaining accuracy. We first conduct triaxial tests to verify that bulk mechanical properties are preserved under local particle refinement. Then, we perform pressure-sinkage and shear-displacement tests, comparing our method to control simulations with homogeneous particle size. We evaluate 36 different DEM beds with varying aggressiveness in particle refinement. Our results show this approach, depending on refinement aggressiveness, can significantly reduce particle count by 2.3 to 25 times and simulation times by 3.1 to 43 times, with normalized errors ranging from 3.4% to 11%. The approach can keep a high resolution at the soil surface, where it is critical for accurately capturing the interaction while allowing larger particles below the surface. The results demonstrate that substantial computational savings can be achieved without significantly compromising simulation accuracy. This method can enhance the feasibility and efficiency of DEM simulations in terramechanics applications. |
2024
|
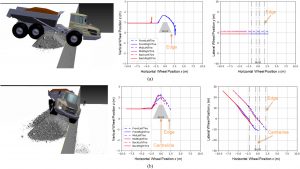 | Thoeni, Klaus; Hartmann, P; Berglund, Tomas; Servin, Martin: Edge protection along haul roads in mines and quarries: A rigorous study based on full-scale testing and numerical modelling. In: Journal of Rock Mechanics and Geotechnical Engineering, 2024, ISBN: 1674-7755. @article{Thoeni2024,
title = {Edge protection along haul roads in mines and quarries: A rigorous study based on full-scale testing and numerical modelling},
author = {Klaus Thoeni and P Hartmann and Tomas Berglund and Martin Servin},
doi = {https://doi.org/10.1016/j.jrmge.2024.10.005},
isbn = {1674-7755},
year = {2024},
date = {2024-10-11},
urldate = {2024-10-11},
journal = {Journal of Rock Mechanics and Geotechnical Engineering},
abstract = {Safety berms (also called safety bunds or windrows), widely employed in surface mining and quarry operations, are typically designed based on rules of thumb. Despite having been used by the industry for more than half a century and accidents happening regularly, their behaviour is still poorly understood. This paper challenges existing practices through a comprehensive investigation combining full-scale experiments and advanced numerical modelling. Focusing on a Volvo A45G articulated dump truck (ADT) and a CAT 773B rigid dump truck (RDT), collision scenarios under various approach conditions and different safety berm geometries and materials are rigorously examined. The calibrated numerical model is used to assess the energy absorption capacity of safety berms with different geometry and to predict a critical velocity for a specific scenario. Back analysis of an actual fatal accident indicated that an ADT adhering to the speed limit could not be stopped by the safety berm designed under current guidelines. The study highlights the importance of considering the entire geometry and the mass and volume of the material used to build the safety berm alongside the speed and approach conditions of the machinery. The findings of the study enable operators to set speed limits based on specific berm geometries or adapt safety berm designs to match speed constraints for specific machinery. This will reduce the risk of fatal accidents and improve haul road safety.},
keywords = {Algoryx, External},
pubstate = {published},
tppubtype = {article}
}
Safety berms (also called safety bunds or windrows), widely employed in surface mining and quarry operations, are typically designed based on rules of thumb. Despite having been used by the industry for more than half a century and accidents happening regularly, their behaviour is still poorly understood. This paper challenges existing practices through a comprehensive investigation combining full-scale experiments and advanced numerical modelling. Focusing on a Volvo A45G articulated dump truck (ADT) and a CAT 773B rigid dump truck (RDT), collision scenarios under various approach conditions and different safety berm geometries and materials are rigorously examined. The calibrated numerical model is used to assess the energy absorption capacity of safety berms with different geometry and to predict a critical velocity for a specific scenario. Back analysis of an actual fatal accident indicated that an ADT adhering to the speed limit could not be stopped by the safety berm designed under current guidelines. The study highlights the importance of considering the entire geometry and the mass and volume of the material used to build the safety berm alongside the speed and approach conditions of the machinery. The findings of the study enable operators to set speed limits based on specific berm geometries or adapt safety berm designs to match speed constraints for specific machinery. This will reduce the risk of fatal accidents and improve haul road safety. |
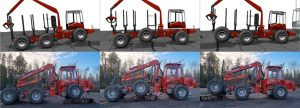 | Wiberg, Viktor; Wallin, Erik; Fälldin, Arvid; Semberg, Tobias; Rossander, Morgan; Wadbro, Eddie; Servin, Martin: Sim-to-real transfer of active suspension control using deep reinforcement learning. In: Robotics and Autonomous Systems, vol. 179, no. 104731, 2024. @article{wiberg2023sim,
title = {Sim-to-real transfer of active suspension control using deep reinforcement learning},
author = {Viktor Wiberg and Erik Wallin and Arvid Fälldin and Tobias Semberg and Morgan Rossander and Eddie Wadbro and Martin Servin},
url = {https://doi.org/10.1016/j.robot.2024.104731
https://arxiv.org/abs/2306.11171
http://umit.cs.umu.se/s2r-ascdrl/},
doi = {10.1016/j.robot.2024.104731},
year = {2024},
date = {2024-07-10},
urldate = {2024-07-10},
journal = {Robotics and Autonomous Systems},
volume = {179},
number = {104731},
abstract = {We explore sim-to-real transfer of deep reinforcement learning controllers for a heavy vehicle with active suspensions designed for traversing rough terrain. While related research primarily focuses on lightweight robots with electric motors and fast actuation, this study uses a forestry vehicle with a complex hydraulic driveline and slow actuation. We simulate the vehicle using multibody dynamics and apply system identification to find an appropriate set of simulation parameters. We then train policies in simulation using various techniques to mitigate the sim-to-real gap, including domain randomization, action delays, and a reward penalty to encourage smooth control. In reality, the policies trained with action delays and a penalty for erratic actions perform nearly at the same level as in simulation. In experiments on level ground, the motion trajectories closely overlap when turning to either side, as well as in a route tracking scenario. When faced with a ramp that requires active use of the suspensions, the simulated and real motions are in close alignment. This shows that the actuator model together with system identification yields a sufficiently accurate model of the actuators. We observe that policies trained without the additional action penalty exhibit fast switching or bang–bang control. These present smooth motions and high performance in simulation but transfer poorly to reality. We find that policies make marginal use of the local height map for perception, showing no indications of predictive planning. However, the strong transfer capabilities entail that further development concerning perception and performance can be largely confined to simulation.},
keywords = {External},
pubstate = {published},
tppubtype = {article}
}
We explore sim-to-real transfer of deep reinforcement learning controllers for a heavy vehicle with active suspensions designed for traversing rough terrain. While related research primarily focuses on lightweight robots with electric motors and fast actuation, this study uses a forestry vehicle with a complex hydraulic driveline and slow actuation. We simulate the vehicle using multibody dynamics and apply system identification to find an appropriate set of simulation parameters. We then train policies in simulation using various techniques to mitigate the sim-to-real gap, including domain randomization, action delays, and a reward penalty to encourage smooth control. In reality, the policies trained with action delays and a penalty for erratic actions perform nearly at the same level as in simulation. In experiments on level ground, the motion trajectories closely overlap when turning to either side, as well as in a route tracking scenario. When faced with a ramp that requires active use of the suspensions, the simulated and real motions are in close alignment. This shows that the actuator model together with system identification yields a sufficiently accurate model of the actuators. We observe that policies trained without the additional action penalty exhibit fast switching or bang–bang control. These present smooth motions and high performance in simulation but transfer poorly to reality. We find that policies make marginal use of the local height map for perception, showing no indications of predictive planning. However, the strong transfer capabilities entail that further development concerning perception and performance can be largely confined to simulation. |
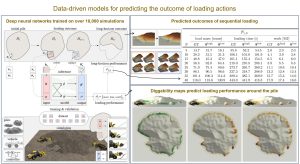 | Aoshima, Koji; Fälldin, Arvid; Wadbro, Eddie; Servin, Martin: World modeling for autonomous wheel loaders. In: Automation, vol. 5, iss. 3, pp. 259-281, 2024. @article{aoshima:2023:pmh,
title = {World modeling for autonomous wheel loaders},
author = {Koji Aoshima and Arvid Fälldin and Eddie Wadbro and Martin Servin},
url = {https://www.mdpi.com/2673-4052/5/3/16
https://www.mdpi.com/2673-4052/5/3/16/pdf
http://umit.cs.umu.se/wl-predictor/},
doi = {10.3390/automation5030016},
year = {2024},
date = {2024-07-07},
urldate = {2023-10-02},
journal = {Automation},
volume = {5},
issue = {3},
pages = {259-281},
abstract = {This paper presents a method for learning world models for wheel loaders performing automatic loading actions on a pile of soil. Data-driven models were learned to output the resulting pile state, loaded mass, time, and work for a single loading cycle given inputs that include a heightmap of the initial pile shape and action parameters for an automatic bucket-filling controller. Long-horizon planning of sequential loading in a dynamically changing environment is thus enabled as repeated model inference. The models, consisting of deep neural networks, were trained on data from a 3D multibody dynamics simulation of over 10,000 random loading actions in gravel piles of different shapes. The accuracy and inference time for predicting the loading performance and the resulting pile state were, on average, 95% in 1.2 ms and 97% in 4.5 ms, respectively. Long-horizon predictions were found feasible over 40 sequential loading actions.},
keywords = {Algoryx, External},
pubstate = {published},
tppubtype = {article}
}
This paper presents a method for learning world models for wheel loaders performing automatic loading actions on a pile of soil. Data-driven models were learned to output the resulting pile state, loaded mass, time, and work for a single loading cycle given inputs that include a heightmap of the initial pile shape and action parameters for an automatic bucket-filling controller. Long-horizon planning of sequential loading in a dynamically changing environment is thus enabled as repeated model inference. The models, consisting of deep neural networks, were trained on data from a 3D multibody dynamics simulation of over 10,000 random loading actions in gravel piles of different shapes. The accuracy and inference time for predicting the loading performance and the resulting pile state were, on average, 95% in 1.2 ms and 97% in 4.5 ms, respectively. Long-horizon predictions were found feasible over 40 sequential loading actions. |
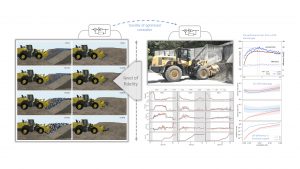 | Aoshima, Koji; Servin, Martin: Examining the simulation-to-reality gap of a wheel loader digging in deformable terrain. In: Multibody System Dynamics, 2024. @article{aoshima2023sim2real,
title = {Examining the simulation-to-reality gap of a wheel loader digging in deformable terrain},
author = {Koji Aoshima and Martin Servin},
url = {https://doi.org/10.1007/s11044-024-10005-5
https://arxiv.org/abs/2310.05765
http://umit.cs.umu.se/wl-sim-to-real/},
doi = {doi.org/10.1007/s11044-024-10005-5},
year = {2024},
date = {2024-06-30},
urldate = {2023-10-10},
journal = {Multibody System Dynamics},
abstract = {We investigate how well a physics-based simulator can replicate a real wheel loader performing bucket filling in a pile of soil. The comparison is made using field test time series of the vehicle motion and actuation forces, loaded mass, and total work. The vehicle was modeled as a rigid multibody system with frictional contacts, driveline, and linear actuators. For the soil, we tested discrete element models of different resolutions, with and without multiscale acceleration. The spatio-temporal resolution ranged between 50-400 mm and 2-500 ms, and the computational speed was between 1/10,000 to 5 times faster than real- time. The simulation-to-reality gap was found to be around 10% and exhibited a weak dependence on the level of fidelity, e.g., compatible with real-time simulation. Furthermore, the sensitivity of an optimized force feedback controller under transfer between different simulation domains was investigated. The domain bias was observed to cause a performance reduction of 5% despite the domain gap being about 15%.},
keywords = {Algoryx, External},
pubstate = {published},
tppubtype = {article}
}
We investigate how well a physics-based simulator can replicate a real wheel loader performing bucket filling in a pile of soil. The comparison is made using field test time series of the vehicle motion and actuation forces, loaded mass, and total work. The vehicle was modeled as a rigid multibody system with frictional contacts, driveline, and linear actuators. For the soil, we tested discrete element models of different resolutions, with and without multiscale acceleration. The spatio-temporal resolution ranged between 50-400 mm and 2-500 ms, and the computational speed was between 1/10,000 to 5 times faster than real- time. The simulation-to-reality gap was found to be around 10% and exhibited a weak dependence on the level of fidelity, e.g., compatible with real-time simulation. Furthermore, the sensitivity of an optimized force feedback controller under transfer between different simulation domains was investigated. The domain bias was observed to cause a performance reduction of 5% despite the domain gap being about 15%. |
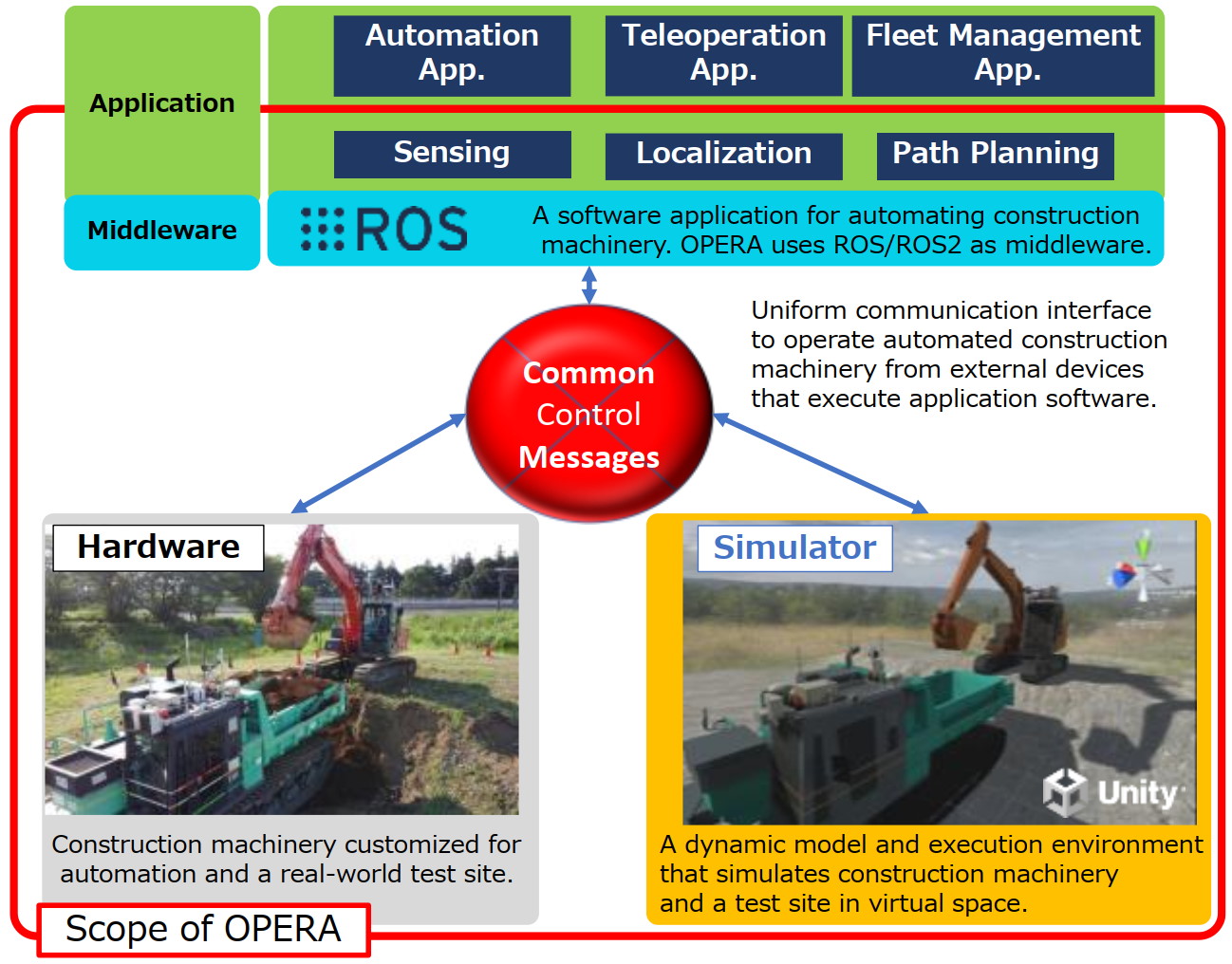 | Endo, Daisuke; Matsusaka, Yosuke; Yamauchi, Genki; Hashimoto, Takeshi: Research on an Open Source Physical Simulator for Autonomous Construction Machinery Development. In: Proceedings of the 41st International Symposium on Automation and Robotics in Construction (ISARC 2024), Lille, France, pp. 1303-1306, International Association for Automation and Robotics in Construction (IAARC), 2024, ISBN: 978-0-6458322-1-1. @inproceedings{nokey,
title = {Research on an Open Source Physical Simulator for Autonomous Construction Machinery Development},
author = {Daisuke Endo and Yosuke Matsusaka and Genki Yamauchi and Takeshi Hashimoto},
url = {https://www.iaarc.org/publications/2024_proceedings_of_the_41st_isarc_lille_france/research_on_an_open_source_physical_simulator_for_autonomous_construction_machinery_development.html
https://www.iaarc.org/publications/fulltext/168_ISARC_2024_Paper_312.pdf},
doi = {10.22260/ISARC2024/0169},
isbn = {978-0-6458322-1-1},
year = {2024},
date = {2024-06-01},
urldate = {2024-06-01},
booktitle = {Proceedings of the 41st International Symposium on Automation and Robotics in Construction (ISARC 2024), Lille, France},
pages = {1303-1306},
publisher = {International Association for Automation and Robotics in Construction (IAARC)},
abstract = {Facing a labor shortage, the civil construction industry is increasingly focused on automating machinery. The Public Works Research Institute in Japan has introduced 'OPERA,' an open development platform, to encourage stakeholder participation in areas like IT, robotics, and AI. Key to OPERA is its open-source software for model-based autonomous construction. Currently, it offers two physical simulators with distinct physics engines, enabling user-specific applications. This paper covers their technical differences, and current states.
},
keywords = {},
pubstate = {published},
tppubtype = {inproceedings}
}
Facing a labor shortage, the civil construction industry is increasingly focused on automating machinery. The Public Works Research Institute in Japan has introduced 'OPERA,' an open development platform, to encourage stakeholder participation in areas like IT, robotics, and AI. Key to OPERA is its open-source software for model-based autonomous construction. Currently, it offers two physical simulators with distinct physics engines, enabling user-specific applications. This paper covers their technical differences, and current states.
|
| Pogilus, Markus; Servin, Martin: Adaptive particle refinement in terramechanical DEM simulation. ISTVS 2024, 21st International and 12th Asia-Pacific Regional Conference of the ISTVS in Yokohama, Oct. 28-31 (2024), 2024. @conference{nokey,
title = {Adaptive particle refinement in terramechanical DEM simulation},
author = {Markus Pogilus and Martin Servin},
url = {http://umit.cs.umu.se/modsimcomplmech/docs/papers/Abstract_Pogilus_2024.pdf},
year = {2024},
date = {2024-05-02},
booktitle = {ISTVS 2024, 21st International and 12th Asia-Pacific Regional Conference of the ISTVS in Yokohama, Oct. 28-31 (2024)},
abstract = {DEM is computationally intensive for granular dynamics simulation, leading to a need for efficient strategies. This study explores using local particle refinement, scaling particle size based on expected spatial resolution needs, inspired by adaptive mesh refinement in FEM. Finer particles are used where intense interaction occurs, and coarser particles further away.
We hypothesize this method can maintain good accuracy while reducing particle count and computational effort. Fine particles are used on the soil bed's top, with coarser particles at greater depth, creating a particle size gradient. By adjusting the gradient we introduce a “scaling aggressiveness”, allowing control over the trade-off between efficiency and accuracy.
We use triaxial tests to verify that the method is scale invariant. Pressure-sinkage and sheardisplacement tests are then used to evaluate the method's effectiveness and accuracy in terramechanics applications. All beds were compared to a reference bed with homogenous particle size, where the mean static sinkage was 1.25 mm for a 50 kPa load. The dynamic sinkage was 73 mm for the full simulation time. For quasi-2D simulations, mild scaling aggressiveness reduced the particle count by 2-4 times with relative error up to 4% for dynamic sinkage (11% for static sinkage). For medium aggressiveness, 4-6 times reduction with relative error of 4% (19% static). For highest aggressiveness, 6-8 times reduction with relative error of 7% (29% static). The internal friction proved to be very resistant to gradient changes, with errors within 1%. When extending the model to full 3D, we estimate up to a reduction in particle count of up to a factor 25.},
keywords = {},
pubstate = {published},
tppubtype = {conference}
}
DEM is computationally intensive for granular dynamics simulation, leading to a need for efficient strategies. This study explores using local particle refinement, scaling particle size based on expected spatial resolution needs, inspired by adaptive mesh refinement in FEM. Finer particles are used where intense interaction occurs, and coarser particles further away.
We hypothesize this method can maintain good accuracy while reducing particle count and computational effort. Fine particles are used on the soil bed's top, with coarser particles at greater depth, creating a particle size gradient. By adjusting the gradient we introduce a “scaling aggressiveness”, allowing control over the trade-off between efficiency and accuracy.
We use triaxial tests to verify that the method is scale invariant. Pressure-sinkage and sheardisplacement tests are then used to evaluate the method's effectiveness and accuracy in terramechanics applications. All beds were compared to a reference bed with homogenous particle size, where the mean static sinkage was 1.25 mm for a 50 kPa load. The dynamic sinkage was 73 mm for the full simulation time. For quasi-2D simulations, mild scaling aggressiveness reduced the particle count by 2-4 times with relative error up to 4% for dynamic sinkage (11% for static sinkage). For medium aggressiveness, 4-6 times reduction with relative error of 4% (19% static). For highest aggressiveness, 6-8 times reduction with relative error of 7% (29% static). The internal friction proved to be very resistant to gradient changes, with errors within 1%. When extending the model to full 3D, we estimate up to a reduction in particle count of up to a factor 25. |
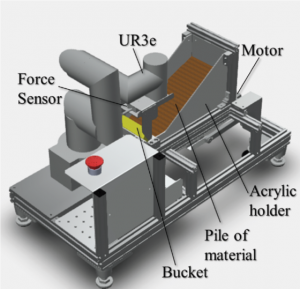 | Koyama, Motoki; Muranaka, Hiroaki; Ishikawa, Masato; Takagi, Yuki: Bayesian Optimization for Digging Control of Wheel-Loader Using Robot Manipulator. In: Journal of Robotics and Mechatronics, vol. 36, no. 2, pp. 273-283, 2024. @article{nokey,
title = {Bayesian Optimization for Digging Control of Wheel-Loader Using Robot Manipulator},
author = {Motoki Koyama and Hiroaki Muranaka and Masato Ishikawa and Yuki Takagi},
url = {https://www.jstage.jst.go.jp/article/jrobomech/36/2/36_273/_pdf},
doi = {https://doi.org/10.20965/jrm.2021.p1248},
year = {2024},
date = {2024-04-20},
urldate = {2024-04-20},
journal = {Journal of Robotics and Mechatronics},
volume = {36},
number = {2},
pages = {273-283},
abstract = {Wheel loaders are construction machines that are mainly used for excavating and loading sedimented ground into dump trucks. The objects to be excavated range from large materials, such as blast rock and crushed stone, to small materials, such as gravel, slag, and coal ash. Therefore, the excavation operation of wheel loaders requires a high skill level to cope with various terrains and soil types. As worker numbers at quarry sites decline, developing highly automated technology to replace operators is crucial. In particular, the geometry of the ground to be excavated by the wheel loader changes with each excavation, so the control parameters must be adapted sequentially during automated excavation. In this study, we proposed an online learning method using Bayesian optimization to search for control parameters from multiple trials and modify them sequentially. In particular, we formulate a multi-objective optimization problem maximizing a weighted linear combination of the payload and workload as an objective function. To validate the proposed method, we constructed an environment in which repeated digging tests can be performed using a robot manipulator with a bucket attached. Through comparative tests between feed-forward control, in which the robot moves along a fixed trajectory independent of the digging reaction force, and off-line control, in which the robot modifies the digging trajectory in response to the digging reaction force, we compared the ability of these methods to cope with terrain volume that is different from that of the optimization trial.},
keywords = {External},
pubstate = {published},
tppubtype = {article}
}
Wheel loaders are construction machines that are mainly used for excavating and loading sedimented ground into dump trucks. The objects to be excavated range from large materials, such as blast rock and crushed stone, to small materials, such as gravel, slag, and coal ash. Therefore, the excavation operation of wheel loaders requires a high skill level to cope with various terrains and soil types. As worker numbers at quarry sites decline, developing highly automated technology to replace operators is crucial. In particular, the geometry of the ground to be excavated by the wheel loader changes with each excavation, so the control parameters must be adapted sequentially during automated excavation. In this study, we proposed an online learning method using Bayesian optimization to search for control parameters from multiple trials and modify them sequentially. In particular, we formulate a multi-objective optimization problem maximizing a weighted linear combination of the payload and workload as an objective function. To validate the proposed method, we constructed an environment in which repeated digging tests can be performed using a robot manipulator with a bucket attached. Through comparative tests between feed-forward control, in which the robot moves along a fixed trajectory independent of the digging reaction force, and off-line control, in which the robot modifies the digging trajectory in response to the digging reaction force, we compared the ability of these methods to cope with terrain volume that is different from that of the optimization trial. |
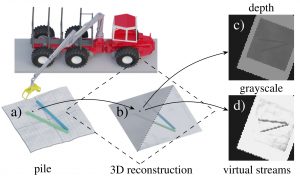 | Wallin, Erik; Wiberg, Viktor; Servin, Martin: Multi-log grasping using reinforcement learning and virtual visual servoing. In: Robotics , vol. 13, iss. 1, no. 3, 2024. @article{wallin2023multi,
title = {Multi-log grasping using reinforcement learning and virtual visual servoing},
author = {Erik Wallin and Viktor Wiberg and Martin Servin},
url = {https://www.mdpi.com/2218-6581/13/1/3
https://arxiv.org/abs/2309.02997
http://umit.cs.umu.se/multi-log-grasping/},
doi = {10.3390/robotics13010003},
year = {2024},
date = {2024-01-30},
urldate = {2023-09-15},
journal = {Robotics },
volume = {13},
number = {3},
issue = {1},
abstract = {We explore multi-log grasping using reinforcement learning and virtual visual servoing for automated forwarding. Automation of forest processes is a major challenge, and many techniques regarding robot control pose different challenges due to the unstructured and harsh outdoor environment. Grasping multiple logs involves problems of dynamics and path planning, where the interaction between the grapple, logs, terrain, and obstacles requires visual information. To address these challenges, we separate image segmentation from crane control and utilize a virtual camera to provide an image stream from 3D reconstructed data. We use Cartesian control to simplify domain transfer. Since log piles are static, visual servoing using a 3D reconstruction of the pile and its surroundings is equivalent to using real camera data until the point of grasping. This relaxes the limit on computational resources and time for the challenge of image segmentation, and allows for collecting data in situations where the log piles are not occluded. The disadvantage is the lack of information during grasping. We demonstrate that this problem is manageable and present an agent that is 95% successful in picking one or several logs from challenging piles of 2-5 logs.},
keywords = {External},
pubstate = {published},
tppubtype = {article}
}
We explore multi-log grasping using reinforcement learning and virtual visual servoing for automated forwarding. Automation of forest processes is a major challenge, and many techniques regarding robot control pose different challenges due to the unstructured and harsh outdoor environment. Grasping multiple logs involves problems of dynamics and path planning, where the interaction between the grapple, logs, terrain, and obstacles requires visual information. To address these challenges, we separate image segmentation from crane control and utilize a virtual camera to provide an image stream from 3D reconstructed data. We use Cartesian control to simplify domain transfer. Since log piles are static, visual servoing using a 3D reconstruction of the pile and its surroundings is equivalent to using real camera data until the point of grasping. This relaxes the limit on computational resources and time for the challenge of image segmentation, and allows for collecting data in situations where the log piles are not occluded. The disadvantage is the lack of information during grasping. We demonstrate that this problem is manageable and present an agent that is 95% successful in picking one or several logs from challenging piles of 2-5 logs. |
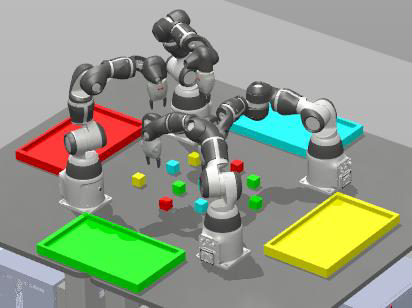 | Mateu-Gomez, Daniel; Martínez-Peral, Francisco José; Perez-Vidal, Carlos: Multi-Arm Trajectory Planning for Optimal Collision-Free Pick-and-Place Operations. In: Technologies, vol. 12, iss. 1, no. 12, 2024. @article{nokey,
title = {Multi-Arm Trajectory Planning for Optimal Collision-Free Pick-and-Place Operations},
author = {Daniel Mateu-Gomez and Francisco José Martínez-Peral and Carlos Perez-Vidal},
url = {https://www.mdpi.com/2227-7080/12/1/12#},
doi = {https://doi.org/10.3390/technologies12010012},
year = {2024},
date = {2024-01-22},
urldate = {2024-01-22},
journal = {Technologies},
volume = {12},
number = {12},
issue = {1},
abstract = {This article addresses the problem of automating a multi-arm pick-and-place robotic system. The objective is to optimize the execution time of a task simultaneously performed by multiple robots, sharing the same workspace, and determining the order of operations to be performed. Due to its ability to address decision-making problems of all kinds, the system is modeled under the mathematical framework of the Markov Decision Process (MDP). In this particular work, the model is adjusted to a deterministic, single-agent, and fully observable system, which allows for its comparison with other resolution methods such as graph search algorithms and Planning Domain Definition Language (PDDL). The proposed approach provides three advantages: it plans the trajectory to perform the task in minimum time; it considers how to avoid collisions between robots; and it automatically generates the robot code for any robot manufacturer and any initial objects’ positions in the workspace. The result meets the objectives and is a fast and robust system that can be safely employed in a production line.},
keywords = {External},
pubstate = {published},
tppubtype = {article}
}
This article addresses the problem of automating a multi-arm pick-and-place robotic system. The objective is to optimize the execution time of a task simultaneously performed by multiple robots, sharing the same workspace, and determining the order of operations to be performed. Due to its ability to address decision-making problems of all kinds, the system is modeled under the mathematical framework of the Markov Decision Process (MDP). In this particular work, the model is adjusted to a deterministic, single-agent, and fully observable system, which allows for its comparison with other resolution methods such as graph search algorithms and Planning Domain Definition Language (PDDL). The proposed approach provides three advantages: it plans the trajectory to perform the task in minimum time; it considers how to avoid collisions between robots; and it automatically generates the robot code for any robot manufacturer and any initial objects’ positions in the workspace. The result meets the objectives and is a fast and robust system that can be safely employed in a production line. |
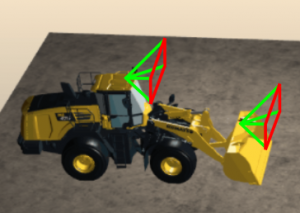 | Lee, Vint; Abbeel, Pieter; Lee, Youngwoon: DreamSmooth: Improving Model-based Reinforcement Learning via Reward Smoothing. In: The Twelfth International Conference on Learning Representations, 2024. @inproceedings{nokey,
title = {DreamSmooth: Improving Model-based Reinforcement Learning via Reward Smoothing},
author = {Vint Lee and Pieter Abbeel and Youngwoon Lee},
url = {https://openreview.net/forum?id=GruDNzQ4ux
https://arxiv.org/pdf/2311.01450.pdf},
year = {2024},
date = {2024-01-16},
urldate = {2024-01-16},
booktitle = {The Twelfth International Conference on Learning Representations},
abstract = {Model-based reinforcement learning (MBRL) has gained much attention for its ability to learn complex behaviors in a sample-efficient way: planning actions by generating imaginary trajectories with predicted rewards. Despite its success, we found that surprisingly, reward prediction is often a bottleneck of MBRL, especially for sparse rewards that are challenging (or even ambiguous) to predict. Motivated by the intuition that humans can learn from rough reward estimates, we propose a simple yet effective reward smoothing approach, DreamSmooth, which learns to predict a temporally-smoothed reward, instead of the exact reward at the given timestep. We empirically show that DreamSmooth achieves state-of-the-art performance on long-horizon sparse-reward tasks both in sample efficiency and final performance without losing performance on common benchmarks, such as Deepmind Control Suite and Atari benchmarks.},
keywords = {},
pubstate = {published},
tppubtype = {inproceedings}
}
Model-based reinforcement learning (MBRL) has gained much attention for its ability to learn complex behaviors in a sample-efficient way: planning actions by generating imaginary trajectories with predicted rewards. Despite its success, we found that surprisingly, reward prediction is often a bottleneck of MBRL, especially for sparse rewards that are challenging (or even ambiguous) to predict. Motivated by the intuition that humans can learn from rough reward estimates, we propose a simple yet effective reward smoothing approach, DreamSmooth, which learns to predict a temporally-smoothed reward, instead of the exact reward at the given timestep. We empirically show that DreamSmooth achieves state-of-the-art performance on long-horizon sparse-reward tasks both in sample efficiency and final performance without losing performance on common benchmarks, such as Deepmind Control Suite and Atari benchmarks. |
2023
|
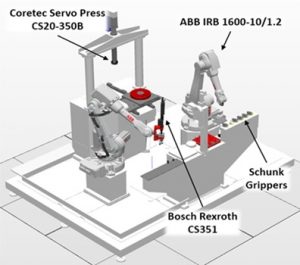 | Raffaeli, Roberto; Neri, Federico; Peruzzini, Margherita; Berselli, Giovanni; Pellicciari, Marcello: Advanced virtual prototyping of robotic cells using physics-based simulation. In: International Journal on Interactive Design and Manufacturing, vol. 18, pp. 981–996, 2023. @article{,
title = {Advanced virtual prototyping of robotic cells using physics-based simulation},
author = {Roberto Raffaeli and Federico Neri and Margherita Peruzzini and Giovanni Berselli and Marcello Pellicciari},
doi = {https://doi.org/10.1007/s12008-023-01677-y},
year = {2023},
date = {2023-12-19},
urldate = {2023-12-19},
journal = {International Journal on Interactive Design and Manufacturing},
volume = {18},
pages = {981–996},
abstract = {Robotic cells are complex mechatronic systems whose final performance is determined by the interaction of the control logics with the mechanical behavior of the process. In this context it is fundamental to develop engineering methods and tools for the virtual prototyping of the cells that emulate both contributions. With such mechatronic digital models, it would be possible to replicate the real behavior of the systems and to optimize the cell productivity, up to building complete digital twins. This paper proposes an engineering method to develop realistic Virtual Prototypes of robotic cells including their geometry, operating logic, performance, and physical behavior. A case study on a robotic cell composed of two anthropomorphic robots for the flexible process of automatic assembly of industrial parts is presented to demonstrate the approach.},
keywords = {External},
pubstate = {published},
tppubtype = {article}
}
Robotic cells are complex mechatronic systems whose final performance is determined by the interaction of the control logics with the mechanical behavior of the process. In this context it is fundamental to develop engineering methods and tools for the virtual prototyping of the cells that emulate both contributions. With such mechatronic digital models, it would be possible to replicate the real behavior of the systems and to optimize the cell productivity, up to building complete digital twins. This paper proposes an engineering method to develop realistic Virtual Prototypes of robotic cells including their geometry, operating logic, performance, and physical behavior. A case study on a robotic cell composed of two anthropomorphic robots for the flexible process of automatic assembly of industrial parts is presented to demonstrate the approach. |
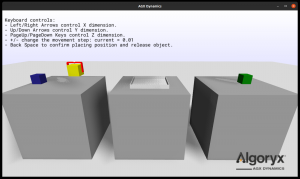 | Iovino, Matteo; Styrud, Jonathan; Smith, Pietro Falco; Christian: A Framework for Learning Behavior Trees in Collaborative Robotic Applications. In: 2023 IEEE 19th International Conference on Automation Science and Engineering (CASE), pp. 1-8, 2023. @inproceedings{nokey,
title = {A Framework for Learning Behavior Trees in Collaborative Robotic Applications},
author = {Matteo Iovino and Jonathan Styrud and Pietro Falco; Christian Smith},
doi = {https://doi.org/10.1109/CASE56687.2023.10260363},
year = {2023},
date = {2023-09-28},
urldate = {2023-09-28},
booktitle = {2023 IEEE 19th International Conference on Automation Science and Engineering (CASE)},
pages = {1-8},
abstract = {In modern industrial collaborative robotic applications, it is desirable to create robot programs automatically, intuitively, and time-efficiently. Moreover, robots need to be controlled by reactive policies to face the unpredictability of the environment they operate in. In this paper we propose a framework that combines a method that learns Behavior Trees (BTs) from demonstration with a method that evolves them with Genetic Programming (GP) for collaborative robotic applications. The main contribution of this paper is to show that by combining the two learning methods we obtain a method that allows non-expert users to semi-automatically, time-efficiently, and interactively generate BTs. We validate the framework with a series of manipulation experiments. The BT is fully learnt in simulation and then transferred to a real collaborative robot.},
keywords = {External},
pubstate = {published},
tppubtype = {inproceedings}
}
In modern industrial collaborative robotic applications, it is desirable to create robot programs automatically, intuitively, and time-efficiently. Moreover, robots need to be controlled by reactive policies to face the unpredictability of the environment they operate in. In this paper we propose a framework that combines a method that learns Behavior Trees (BTs) from demonstration with a method that evolves them with Genetic Programming (GP) for collaborative robotic applications. The main contribution of this paper is to show that by combining the two learning methods we obtain a method that allows non-expert users to semi-automatically, time-efficiently, and interactively generate BTs. We validate the framework with a series of manipulation experiments. The BT is fully learnt in simulation and then transferred to a real collaborative robot. |
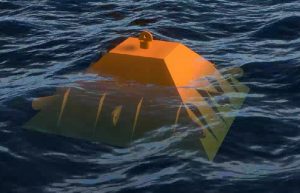 | Martinez, Rodrigo; Arnau, Sergi; Scullion, Callum; Collins, Paddy; Neilson, Richard D; Kapitaniak, Marcin: Variable buoyancy anchor deployment analysis for floating wind applications using a Marine Simulator. In: Ocean Engineering, vol. 285, pp. 115417, 2023, ISSN: 0029-8018. @article{MARTINEZ2023115417,
title = {Variable buoyancy anchor deployment analysis for floating wind applications using a Marine Simulator},
author = {Rodrigo Martinez and Sergi Arnau and Callum Scullion and Paddy Collins and Richard D Neilson and Marcin Kapitaniak},
url = {https://www.sciencedirect.com/science/article/pii/S0029801823018012},
doi = {https://doi.org/10.1016/j.oceaneng.2023.115417},
issn = {0029-8018},
year = {2023},
date = {2023-07-16},
journal = {Ocean Engineering},
volume = {285},
pages = {115417},
abstract = {To study the feasibility of deploying a novel type of anchor with variable buoyancy for mooring floating offshore wind turbines, a set of detailed modelling studies was performed in the state of-the-art Marine Simulator at the National Decommissioning Centre. The aim of the multiphysics simulations is to assess fully a proposed craneless deployment method that involves towing the anchor from the harbour to the installation site, pumping liquid ballast to overcome anchor’s buoyancy and lowering it to the seabed using only a winch, thereby simplifying the process, and reducing installation costs. As a test case, a novel shape of the floating anchor is considered, to establish the feasibility of its deployment in conjunction with the variable buoyancy technology and installation sequence. The analysis is divided into three sections: characterisation of the anchor buoyancy, positioning the anchor under the stern of the vessel and the controlled descent of the anchor to the seabed, under varying weather and operational conditions (e.g significant wave height, current, winch velocity, liquid ballast mass, ballast pump rate). The analysis allows assessment of the importance of the different factors affecting the proposed deployment scenario of variable buoyancy anchors, such as the winch velocity, the ballast mass and the pump rate.},
keywords = {External},
pubstate = {published},
tppubtype = {article}
}
To study the feasibility of deploying a novel type of anchor with variable buoyancy for mooring floating offshore wind turbines, a set of detailed modelling studies was performed in the state of-the-art Marine Simulator at the National Decommissioning Centre. The aim of the multiphysics simulations is to assess fully a proposed craneless deployment method that involves towing the anchor from the harbour to the installation site, pumping liquid ballast to overcome anchor’s buoyancy and lowering it to the seabed using only a winch, thereby simplifying the process, and reducing installation costs. As a test case, a novel shape of the floating anchor is considered, to establish the feasibility of its deployment in conjunction with the variable buoyancy technology and installation sequence. The analysis is divided into three sections: characterisation of the anchor buoyancy, positioning the anchor under the stern of the vessel and the controlled descent of the anchor to the seabed, under varying weather and operational conditions (e.g significant wave height, current, winch velocity, liquid ballast mass, ballast pump rate). The analysis allows assessment of the importance of the different factors affecting the proposed deployment scenario of variable buoyancy anchors, such as the winch velocity, the ballast mass and the pump rate. |
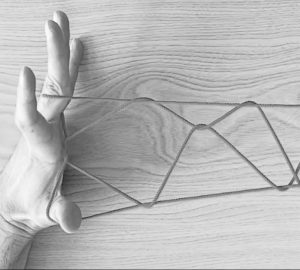 | Nishita, Seikoh: String Figure Simulation with Multiresolution Wire Model. In: Proceedings of the 2023 12th International Conference on Software and Computer Applications, pp. 65–70, Association for Computing Machinery, New York, NY, USA, 2023, ISBN: 9781450398589. @inproceedings{nokey,
title = {String Figure Simulation with Multiresolution Wire Model},
author = {Seikoh Nishita},
doi = {https://doi.org/10.1145/3587828.3587839},
isbn = {9781450398589},
year = {2023},
date = {2023-06-20},
urldate = {2023-06-20},
booktitle = {Proceedings of the 2023 12th International Conference on Software and Computer Applications},
pages = {65–70},
publisher = {Association for Computing Machinery},
address = {New York, NY, USA},
series = {ICSCA '23},
abstract = {String figure is a traditional game with a loop of a string played by hooking and/or unhooking strands of the loop from fingers to produce patterns representing certain objects. The patterns of the string figure change dynamically by the string manipulations by fingers. A computer-based method based on knot theory has been proposed to make string figure patterns. This method represents a string in the string figure as an extended knot diagram and generates string figure patterns by monotonically decreasing the number of crossing points. However, there are string figures where this method does not make the patterns correctly. In contrast, this paper proposes a method using physical simulation from the viewpoint that the string figure patterns are determined according to the tension and frictional force applied to the string. To evaluate the proposed method, we conducted two types of experiments. In the first experiments, we showed that physical simulations using an adaptive multi-resolution wire model have sufficient capability to compute the string figure patterns. In the second, we conducted experiments to make patterns for instances of the string figure. The experimental results indicate that the proposed method can correctly make the string figure patterns in most cases. We also found that the proposed method can make some of the string figure patterns that cannot be generated by conventional methods.},
keywords = {External},
pubstate = {published},
tppubtype = {inproceedings}
}
String figure is a traditional game with a loop of a string played by hooking and/or unhooking strands of the loop from fingers to produce patterns representing certain objects. The patterns of the string figure change dynamically by the string manipulations by fingers. A computer-based method based on knot theory has been proposed to make string figure patterns. This method represents a string in the string figure as an extended knot diagram and generates string figure patterns by monotonically decreasing the number of crossing points. However, there are string figures where this method does not make the patterns correctly. In contrast, this paper proposes a method using physical simulation from the viewpoint that the string figure patterns are determined according to the tension and frictional force applied to the string. To evaluate the proposed method, we conducted two types of experiments. In the first experiments, we showed that physical simulations using an adaptive multi-resolution wire model have sufficient capability to compute the string figure patterns. In the second, we conducted experiments to make patterns for instances of the string figure. The experimental results indicate that the proposed method can correctly make the string figure patterns in most cases. We also found that the proposed method can make some of the string figure patterns that cannot be generated by conventional methods. |
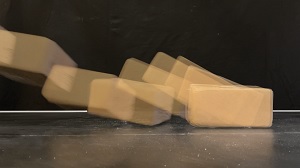 | Jongeneel, Maarten Johannes; Poort, Luuk; van de Wouw, Nathan; Saccon, Alessandro: Experimental Validation of Nonsmooth Dynamics Simulations for Robotic Tossing involving Friction and Impacts. 2023, (working paper or preprint). @unpublished{jongeneel:hal-03974604,
title = {Experimental Validation of Nonsmooth Dynamics Simulations for Robotic Tossing involving Friction and Impacts},
author = {Maarten Johannes Jongeneel and Luuk Poort and Nathan van de Wouw and Alessandro Saccon},
url = {https://hal.science/hal-03974604},
year = {2023},
date = {2023-02-23},
abstract = {In this paper, we evaluate the prediction performance of two nonsmooth rigid-body dynamics simulators on realworld data with spatial impacts in the context of robotic tossing and visual tracking. We perform a parameter identification procedure to find the coefficient of friction and restitution of different objects via a velocity-based and trajectory-based cost function. Our results show that these two identification criteria lead to different parameter values, and these criteria should be chosen in consideration of the application at hand. We compare the simulated predicted rest-pose with measurement data and perform a sensitivity analysis to assess how uncertainty on the identified parameters affects the rest-pose prediction of the object. For the robotic tossing application at hand, we show that the rest-pose prediction is insensitive to the coefficient of restitution, and accurate predictions are obtained via simulations using only ballistic motion and friction.},
note = {working paper or preprint},
keywords = {External},
pubstate = {published},
tppubtype = {unpublished}
}
In this paper, we evaluate the prediction performance of two nonsmooth rigid-body dynamics simulators on realworld data with spatial impacts in the context of robotic tossing and visual tracking. We perform a parameter identification procedure to find the coefficient of friction and restitution of different objects via a velocity-based and trajectory-based cost function. Our results show that these two identification criteria lead to different parameter values, and these criteria should be chosen in consideration of the application at hand. We compare the simulated predicted rest-pose with measurement data and perform a sensitivity analysis to assess how uncertainty on the identified parameters affects the rest-pose prediction of the object. For the robotic tossing application at hand, we show that the rest-pose prediction is insensitive to the coefficient of restitution, and accurate predictions are obtained via simulations using only ballistic motion and friction. |
2022
|
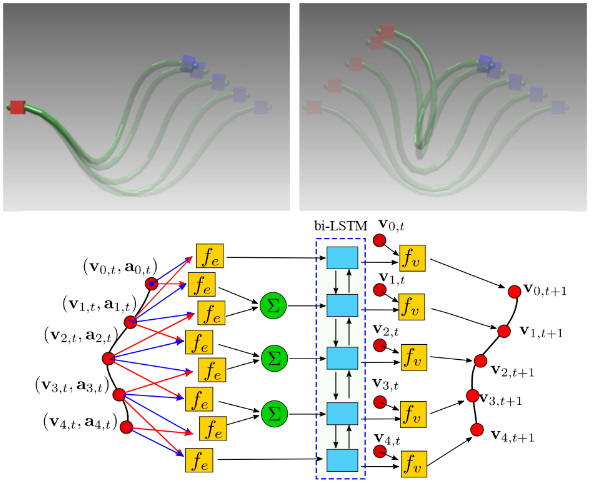 | Yang, Yuxuan; Stork, Johannes A.; Stoyanov, Todor: Online Model Learning for Shape Control of Deformable Linear Objects. In: 2022 IEEE/RSJ International Conference on Intelligent Robots and Systems (IROS), pp. 4056-4062, 2022. @article{yang2022,
title = {Online Model Learning for Shape Control of Deformable Linear Objects},
author = {Yuxuan Yang and Johannes A. Stork and Todor Stoyanov},
url = {https://ieeexplore.ieee.org/document/9981080},
doi = {10.1109/IROS47612.2022.9981080},
year = {2022},
date = {2022-12-26},
journal = {2022 IEEE/RSJ International Conference on Intelligent Robots and Systems (IROS)},
pages = {4056-4062},
abstract = {Traditional approaches to manipulating the state of deformable linear objects (DLOs) - i.e., cables, ropes - rely on model-based planning. However, constructing an accurate dynamic model of a DLO is challenging due to the complexity of interactions and a high number of degrees of freedom. This renders the task of achieving a desired DLO shape particularly difficult and motivates the use of model-free alternatives, which while maintaining generality suffer from a high sample complexity. In this paper, we bridge the gap between these fundamentally different approaches and propose a framework that learns dynamic models of DLOs through trial-and-error interaction. Akin to model-based reinforcement learning (RL), we interleave learning and exploration to solve a 3D shape control task for a DLO. Our approach requires only a fraction of the interaction samples of the current state-of-the-art model-free RL alternatives to achieve superior shape control performance. Unlike offline model learning, our approach does not require expert knowledge for data collection, retains the ability to explore, and automatically selects relevant experience.},
keywords = {External},
pubstate = {published},
tppubtype = {article}
}
Traditional approaches to manipulating the state of deformable linear objects (DLOs) - i.e., cables, ropes - rely on model-based planning. However, constructing an accurate dynamic model of a DLO is challenging due to the complexity of interactions and a high number of degrees of freedom. This renders the task of achieving a desired DLO shape particularly difficult and motivates the use of model-free alternatives, which while maintaining generality suffer from a high sample complexity. In this paper, we bridge the gap between these fundamentally different approaches and propose a framework that learns dynamic models of DLOs through trial-and-error interaction. Akin to model-based reinforcement learning (RL), we interleave learning and exploration to solve a 3D shape control task for a DLO. Our approach requires only a fraction of the interaction samples of the current state-of-the-art model-free RL alternatives to achieve superior shape control performance. Unlike offline model learning, our approach does not require expert knowledge for data collection, retains the ability to explore, and automatically selects relevant experience. |
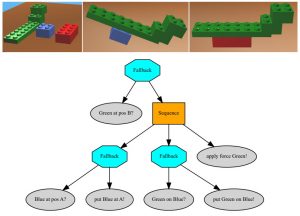 | Styrud, Jonathan; Iovino, Matteo; Norrlöf, Mikael; Björkman, Mårten; Smith, Christian: Combining planning and learning of behavior trees for robotic assembly. In: 2022 International Conference on Robotics and Automation (ICRA), pp. 11511–11517, IEEE, 2022. @inproceedings{styrud2022combining,
title = {Combining planning and learning of behavior trees for robotic assembly},
author = {Styrud, Jonathan and Iovino, Matteo and Norrlöf, Mikael and Björkman, Mårten and Smith, Christian},
url = {https://ieeexplore.ieee.org/abstract/document/9812086
https://ieeexplore.ieee.org/stamp/stamp.jsp?tp=&arnumber=9812086},
doi = {10.1109/ICRA46639.2022.9812086},
year = {2022},
date = {2022-10-01},
booktitle = {2022 International Conference on Robotics and Automation (ICRA)},
pages = {11511--11517},
publisher = {IEEE},
abstract = {Industrial robots can solve tasks in controlled environments, but modern applications require robots able to operate also in unpredictable surroundings. An increasingly popular reactive policy architecture in robotics is Behavior Trees (BTs) but as other architectures, programming time drives cost and limits flexibility. The two main branches of algorithms to generate policies automatically, automated planning and machine learning, both have their own drawbacks and have not previously been combined for generation of BTs. We propose a method for creating BTs by combining these branches, inserting the result of an automated planner into the population of a Genetic Programming algorithm. Experiments confirm that the proposed method performs well on a variety of robotic assembly problems and outperforms the base methods used separately. We also show that this high level learning of Behavior Trees can be transferred to a real system without further training.},
keywords = {External},
pubstate = {published},
tppubtype = {inproceedings}
}
Industrial robots can solve tasks in controlled environments, but modern applications require robots able to operate also in unpredictable surroundings. An increasingly popular reactive policy architecture in robotics is Behavior Trees (BTs) but as other architectures, programming time drives cost and limits flexibility. The two main branches of algorithms to generate policies automatically, automated planning and machine learning, both have their own drawbacks and have not previously been combined for generation of BTs. We propose a method for creating BTs by combining these branches, inserting the result of an automated planner into the population of a Genetic Programming algorithm. Experiments confirm that the proposed method performs well on a variety of robotic assembly problems and outperforms the base methods used separately. We also show that this high level learning of Behavior Trees can be transferred to a real system without further training. |
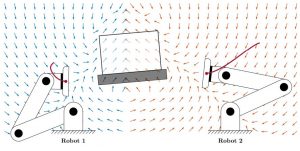 | Cosgun, A; van Steen,: Impact-aware dual-arm grasping through time-invariant reference spreading. In: 2022. @article{cosgunimpact,
title = {Impact-aware dual-arm grasping through time-invariant reference spreading},
author = {Cosgun, A and van Steen},
url = {https://pure.tue.nl/ws/portalfiles/portal/224201404/1020574_Impact_aware_grasping_through_time_invariant_reference_spreading.pdf},
year = {2022},
date = {2022-10-01},
abstract = {Eindhoven University of Technology},
abstract = {In robotic applications which involve handling objects or interaction with the environment,
robots generally establish contact at near-zero speed due to the effect of impacts. At impact, rapid velocity changes occur in the robot joints accompanied by large contact forces,
which can cause damage to the robot, humans, or the environment. As a result, robots
typically accelerate and decelerate repeatedly to achieve a task that involves contact, which
negatively affects the energy consumption and throughput. In order to increase the performance, control strategies are proposed that take into account the impact dynamics, which
are referred to as impact-aware control. Impact-aware control strategies exploit impacts, to
increase the swiftness of the performed tasks and reach a more human-like behavior. One
of the challenges in impact-aware control strategies is that, in presence of uncertainties, a
robot can experience an impact at a different time than expected. This causes the actual
state of the system to reside in a different mode than prescribed by the time-based reference
trajectory, which can cause the robot to compensate aggressively and possibly becoming unstable. Furthermore, when contact is expected to be established at multiple contact points
simultaneously, the controller can enter an unspecified and typically unpredictable mode.
Lastly, in case of external perturbations, the robot can lag behind compared to its reference
state at that time instant, which would cause the robot to “catch up” in order to reach its
desired state, which would generally be undesired.
The combination of a time-invariant control strategies and an impact-aware reference spreading control strategy can provide a possible solution for these challenges. By employing a reference that is solely a function of the robot’s position and orientation, and extending it around
an expected impact position, robots can be controlled towards an intentional impact in the
presence of uncertainties. Therefore, the goal of this project is to design an impact-aware
time-invariant reference spreading control strategy for a dual-arm robotic system capable
of swift grasping. To achieve this, a procedure is proposed to generate time-invariant references that can steer the robots towards a desired position and speed to swiftly grasp an
object, while ensuring the reference is compatible through the impact dynamics. In order
to be applicable on a dual-arm robotic system, the time-invariant reference spreading control strategy is cast into a task-based quadratic-programming control framework, which is
a common framework for control of complex systems. Furthermore, an intermediate mode
control strategy is proposed to ensure full contact is established when a loss of simultaneity
occurs. Additionally, a synchronization strategy is formulated to ensure the robots reach
the object at the same time. Finally, the effectiveness of the proposed control approach is
validated by means of numerical simulation studies on a two-dimensional, and a more complex three-dimensional scenario, which consists of a realistic seven degrees of freedom robot
setup.},
keywords = {External},
pubstate = {published},
tppubtype = {article}
}
Eindhoven University of Technology},
abstract = {In robotic applications which involve handling objects or interaction with the environment,
robots generally establish contact at near-zero speed due to the effect of impacts. At impact, rapid velocity changes occur in the robot joints accompanied by large contact forces,
which can cause damage to the robot, humans, or the environment. As a result, robots
typically accelerate and decelerate repeatedly to achieve a task that involves contact, which
negatively affects the energy consumption and throughput. In order to increase the performance, control strategies are proposed that take into account the impact dynamics, which
are referred to as impact-aware control. Impact-aware control strategies exploit impacts, to
increase the swiftness of the performed tasks and reach a more human-like behavior. One
of the challenges in impact-aware control strategies is that, in presence of uncertainties, a
robot can experience an impact at a different time than expected. This causes the actual
state of the system to reside in a different mode than prescribed by the time-based reference
trajectory, which can cause the robot to compensate aggressively and possibly becoming unstable. Furthermore, when contact is expected to be established at multiple contact points
simultaneously, the controller can enter an unspecified and typically unpredictable mode.
Lastly, in case of external perturbations, the robot can lag behind compared to its reference
state at that time instant, which would cause the robot to “catch up” in order to reach its
desired state, which would generally be undesired.
The combination of a time-invariant control strategies and an impact-aware reference spreading control strategy can provide a possible solution for these challenges. By employing a reference that is solely a function of the robot’s position and orientation, and extending it around
an expected impact position, robots can be controlled towards an intentional impact in the
presence of uncertainties. Therefore, the goal of this project is to design an impact-aware
time-invariant reference spreading control strategy for a dual-arm robotic system capable
of swift grasping. To achieve this, a procedure is proposed to generate time-invariant references that can steer the robots towards a desired position and speed to swiftly grasp an
object, while ensuring the reference is compatible through the impact dynamics. In order
to be applicable on a dual-arm robotic system, the time-invariant reference spreading control strategy is cast into a task-based quadratic-programming control framework, which is
a common framework for control of complex systems. Furthermore, an intermediate mode
control strategy is proposed to ensure full contact is established when a loss of simultaneity
occurs. Additionally, a synchronization strategy is formulated to ensure the robots reach
the object at the same time. Finally, the effectiveness of the proposed control approach is
validated by means of numerical simulation studies on a two-dimensional, and a more complex three-dimensional scenario, which consists of a realistic seven degrees of freedom robot
setup. |
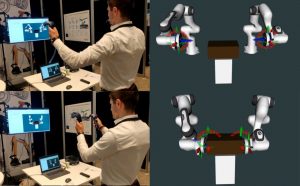 | van Oorschot, S. P. M.: Design and numerical validation of a dual-arm telerobotic simulation for object grabbing. Eindhoven University of Technology, 2022. @mastersthesis{Oorschot2022,
title = {Design and numerical validation of a dual-arm telerobotic simulation for object grabbing},
author = {van Oorschot, S.P.M.},
url = {https://pure.tue.nl/ws/portalfiles/portal/211664977/1014209_Oorschot.pdf},
year = {2022},
date = {2022-10-01},
school = {Eindhoven University of Technology},
abstract = {Currently, most robots in the parcel industry manipulate objects by making contact with a
near-zero velocity, limiting their movement to pick-and-place actions. The incorporation of
impacts, i.e., making contact with non-zero velocity, in the control of robots can extend the
robot’s actions with grabbing, which improves the throughput of such systems.
The control of robots during impact requires the use of novel control algorithms, for which
an estimation of the robot’s impact behavior is required. The application and development
of such control algorithms, and the study towards robot impacts in order to improve such
estimations are both subjected to the impulsive nature of impacts, and the complications
that arise consequentially. First of all, impacting with robots poses a safety hazard for both
the operator, the robot, and the environment, as impacts are often paired with quick changes
in velocity, peaking forces, and peaking torques. Second of all, the generation of reference
trajectories for robotic grabbing is time-consuming and undextrous, which raises the need
for a more human-oriented method of instructing robot reference trajectories.
In this work, a software framework is developed which aims at tackling both of the aforementioned problems. This framework combines the human capability of trajectory planning
and of performing swift grabbing actions by controlling the robot setup via teleoperation.
The teleoperated reference trajectories control a QP controller, which interacts with a simulation of the robot scene which mimics real life physics, allowing preliminary testing to be
conducted on this virtual system. Experiments are conducted to test the usability of the teleoperation system. Furthermore, to illustrate that the system can indeed aid in development
of novel control techniques and impact behavior estimations, experiments are conducted
which focus on the effect of the robot posture and the end effector impact location on the
impact behavior of the system. Results show that the developed system is intuitive to use,
and that the robot elbow pose and the location of the impact have a significant effect on the
robot’s impact behavior.},
keywords = {External},
pubstate = {published},
tppubtype = {mastersthesis}
}
Currently, most robots in the parcel industry manipulate objects by making contact with a
near-zero velocity, limiting their movement to pick-and-place actions. The incorporation of
impacts, i.e., making contact with non-zero velocity, in the control of robots can extend the
robot’s actions with grabbing, which improves the throughput of such systems.
The control of robots during impact requires the use of novel control algorithms, for which
an estimation of the robot’s impact behavior is required. The application and development
of such control algorithms, and the study towards robot impacts in order to improve such
estimations are both subjected to the impulsive nature of impacts, and the complications
that arise consequentially. First of all, impacting with robots poses a safety hazard for both
the operator, the robot, and the environment, as impacts are often paired with quick changes
in velocity, peaking forces, and peaking torques. Second of all, the generation of reference
trajectories for robotic grabbing is time-consuming and undextrous, which raises the need
for a more human-oriented method of instructing robot reference trajectories.
In this work, a software framework is developed which aims at tackling both of the aforementioned problems. This framework combines the human capability of trajectory planning
and of performing swift grabbing actions by controlling the robot setup via teleoperation.
The teleoperated reference trajectories control a QP controller, which interacts with a simulation of the robot scene which mimics real life physics, allowing preliminary testing to be
conducted on this virtual system. Experiments are conducted to test the usability of the teleoperation system. Furthermore, to illustrate that the system can indeed aid in development
of novel control techniques and impact behavior estimations, experiments are conducted
which focus on the effect of the robot posture and the end effector impact location on the
impact behavior of the system. Results show that the developed system is intuitive to use,
and that the robot elbow pose and the location of the impact have a significant effect on the
robot’s impact behavior. |
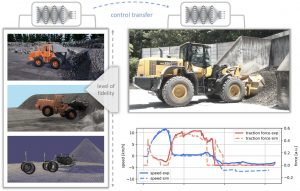 | Aoshima, Koji; Lindmark, Daniel; Servin, Martin: Examining the simulation-to-reality-gap of a wheel loader interacting with deformable terrain. 11th Asia-Pacific Regional Conference of the ISTVS September 26-28, 2022, Harbin, China, 2022. @conference{aoshima2022examining,
title = {Examining the simulation-to-reality-gap of a wheel loader interacting with deformable terrain},
author = {Aoshima, Koji and Lindmark, Daniel and Servin, Martin},
url = {http://umit.cs.umu.se/wl-sim2real/},
year = {2022},
date = {2022-09-01},
booktitle = {11th Asia-Pacific Regional Conference of the ISTVS September 26-28, 2022, Harbin, China},
abstract = {Simulators are essential for developing autonomous control of off-road vehicles and heavy equipment. They allow automatic testing under safe and controllable conditions, and the generation of large amounts of synthetic and annotated training data necessary for deep learning to be applied [1]. Limiting factors are the computational speed and how accurately the simulator reflects the real system. When the deviation is too large, a controller transfers poorly from the simulated to the real environment. On the other hand, a finely resolved simulator easily becomes too computationally intense and slow for running the necessary number of simulations or keeping realtime pace with hardware in the loop. We investigate how well a physics-based simulator can be made to match its physical counterpart, a full-scale wheel loader instrumented with motion and force sensors performing a bucket filling operation [2]. The simulated vehicle is represented as a rigid multibody system with nonsmooth contact and driveline dynamics. The terrain model combines descriptions of the frictional-cohesive soil as a continuous solid and particles, discretized in voxels and discrete elements [3]. Strong and stable force coupling with the equipment is mediated via rigid aggregate bodies capturing the bulk mechanics of the soil. The results include analysis of the agreement between a calibrated simulation model and the field tests, and of how the simulation performance and accuracy depend on spatial and temporal resolution. The system’s degrees of freedom range from hundreds to millions and the simulation speed up to ten times faster than realtime. Furthermore, it is investigated how sensitive a deep learning controller is to variations in the simulator environment parameters.},
keywords = {External},
pubstate = {published},
tppubtype = {conference}
}
Simulators are essential for developing autonomous control of off-road vehicles and heavy equipment. They allow automatic testing under safe and controllable conditions, and the generation of large amounts of synthetic and annotated training data necessary for deep learning to be applied [1]. Limiting factors are the computational speed and how accurately the simulator reflects the real system. When the deviation is too large, a controller transfers poorly from the simulated to the real environment. On the other hand, a finely resolved simulator easily becomes too computationally intense and slow for running the necessary number of simulations or keeping realtime pace with hardware in the loop. We investigate how well a physics-based simulator can be made to match its physical counterpart, a full-scale wheel loader instrumented with motion and force sensors performing a bucket filling operation [2]. The simulated vehicle is represented as a rigid multibody system with nonsmooth contact and driveline dynamics. The terrain model combines descriptions of the frictional-cohesive soil as a continuous solid and particles, discretized in voxels and discrete elements [3]. Strong and stable force coupling with the equipment is mediated via rigid aggregate bodies capturing the bulk mechanics of the soil. The results include analysis of the agreement between a calibrated simulation model and the field tests, and of how the simulation performance and accuracy depend on spatial and temporal resolution. The system’s degrees of freedom range from hundreds to millions and the simulation speed up to ten times faster than realtime. Furthermore, it is investigated how sensitive a deep learning controller is to variations in the simulator environment parameters. |
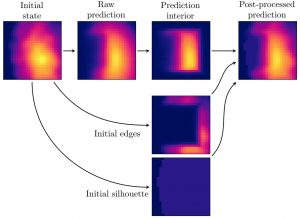 | Fälldin, Arvid: Learning by Digging: A Differentiable Prediction Model for an Autonomous Wheel Loader. Department of Physics, Umeå University, 2022. @mastersthesis{Falldin2022,
title = {Learning by Digging: A Differentiable Prediction Model for an Autonomous Wheel Loader},
author = {Arvid Fälldin},
url = {http://urn.kb.se/resolve?urn=urn:nbn:se:umu:diva-197165
http://www.diva-portal.org/smash/get/diva2:1675538/FULLTEXT01.pdf},
year = {2022},
date = {2022-06-22},
school = {Department of Physics, Umeå University},
abstract = {Wheel loaders are heavy duty machines that are ubiquitous on construction sites and in mines all over the world. Fully autonomous wheel loaders remains an open problem but the industry is hoping that increasing their level of autonomy will help to reduce costs and energy consumption while also increasing workplace safety. Operating a wheel loader efficiently requires dig plans that extend over multiple dig cycles and not just one at a time. This calls for a model that can predict both the performance of a dig action and the resulting shape of the pile. In this thesis project, we use simulations to develop a data-driven artificial neural network model that can predict the outcome of a dig action. The model is able to predict the wheel loader’s productivity with an average error of 7.3%, and the altered shape of the pile with an average relative error of 4.5%. We also show that automatic differentiation techniques can be used to accurately differentiate the model with respect to input. This makes it possible to use gradient-based optimization methods to find the dig action that maximises the performance of the wheel loader.},
keywords = {External},
pubstate = {published},
tppubtype = {mastersthesis}
}
Wheel loaders are heavy duty machines that are ubiquitous on construction sites and in mines all over the world. Fully autonomous wheel loaders remains an open problem but the industry is hoping that increasing their level of autonomy will help to reduce costs and energy consumption while also increasing workplace safety. Operating a wheel loader efficiently requires dig plans that extend over multiple dig cycles and not just one at a time. This calls for a model that can predict both the performance of a dig action and the resulting shape of the pile. In this thesis project, we use simulations to develop a data-driven artificial neural network model that can predict the outcome of a dig action. The model is able to predict the wheel loader’s productivity with an average error of 7.3%, and the altered shape of the pile with an average relative error of 4.5%. We also show that automatic differentiation techniques can be used to accurately differentiate the model with respect to input. This makes it possible to use gradient-based optimization methods to find the dig action that maximises the performance of the wheel loader. |
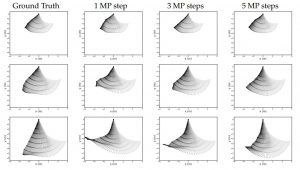 | Marklund, Hannes: Expressibility of multiscale physics in deep networks. Department of Physics, Umeå University, 2022. @mastersthesis{itembibentry{marklund2022expressibility},
title = {Expressibility of multiscale physics in deep networks},
author = {Hannes Marklund},
url = {https://umu.diva-portal.org/smash/record.jsf?pid=diva2:1678353},
year = {2022},
date = {2022-06-10},
school = {Department of Physics, Umeå University},
abstract = {Motivated by the successes in the field of deep learning, the scientific community has been increasingly interested in neural networks that are able to reason about physics. As neural networks are universal approximators, they could in theory learn representations that are more efficient than traditional methods whenever improvements are theoretically possible. This thesis, done in collaboration with Algoryx, serves both as a review of the current research in this area and as an experimental investigation of a subset of the proposed methods. We focus on how useful these methods are as textit{learnable simulators} of mechanical systems that are possibly constrained and multiscale. The experimental investigation considers low-dimensional problems with training data generated by either custom numerical integration or by use of the physics engine AGX Dynamics. A good learnable simulator should express some important properties such as being stable, accurate, generalizable, and fast. Importantly, a generalizable simulator must be able to represent reconfigurable environments, requiring a model known as a graph neural network (GNN). The experimental results show that black-box neural networks are limited to approximate physics in the states it has been trained on. The results also suggest that traditional message-passing GNNs have a limited ability to represent more challenging multiscale systems. This is currently the most widely used method to realize GNNs and thus raises concern as there is not much to be gained by investing time into a method with fundamental limitations. },
keywords = {Algoryx},
pubstate = {published},
tppubtype = {mastersthesis}
}
Motivated by the successes in the field of deep learning, the scientific community has been increasingly interested in neural networks that are able to reason about physics. As neural networks are universal approximators, they could in theory learn representations that are more efficient than traditional methods whenever improvements are theoretically possible. This thesis, done in collaboration with Algoryx, serves both as a review of the current research in this area and as an experimental investigation of a subset of the proposed methods. We focus on how useful these methods are as textit{learnable simulators} of mechanical systems that are possibly constrained and multiscale. The experimental investigation considers low-dimensional problems with training data generated by either custom numerical integration or by use of the physics engine AGX Dynamics. A good learnable simulator should express some important properties such as being stable, accurate, generalizable, and fast. Importantly, a generalizable simulator must be able to represent reconfigurable environments, requiring a model known as a graph neural network (GNN). The experimental results show that black-box neural networks are limited to approximate physics in the states it has been trained on. The results also suggest that traditional message-passing GNNs have a limited ability to represent more challenging multiscale systems. This is currently the most widely used method to realize GNNs and thus raises concern as there is not much to be gained by investing time into a method with fundamental limitations. |
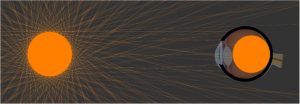 | Özdemir, Erdougan; Coramik, Mustafa: Development of a virtual teaching environment with Algodoo:‘eye’and ‘cactus type light source’models. In: Physics Education, vol. 57, no. 4, pp. 045022, 2022. @article{ozdemir2022development,
title = {Development of a virtual teaching environment with Algodoo:‘eye’and ‘cactus type light source’models},
author = {Özdemir, Erdougan and Coramik, Mustafa},
url = {https://iopscience.iop.org/article/10.1088/1361-6552/ac60b0/pdf},
year = {2022},
date = {2022-05-01},
journal = {Physics Education},
volume = {57},
number = {4},
pages = {045022},
keywords = {External},
pubstate = {published},
tppubtype = {article}
}
|
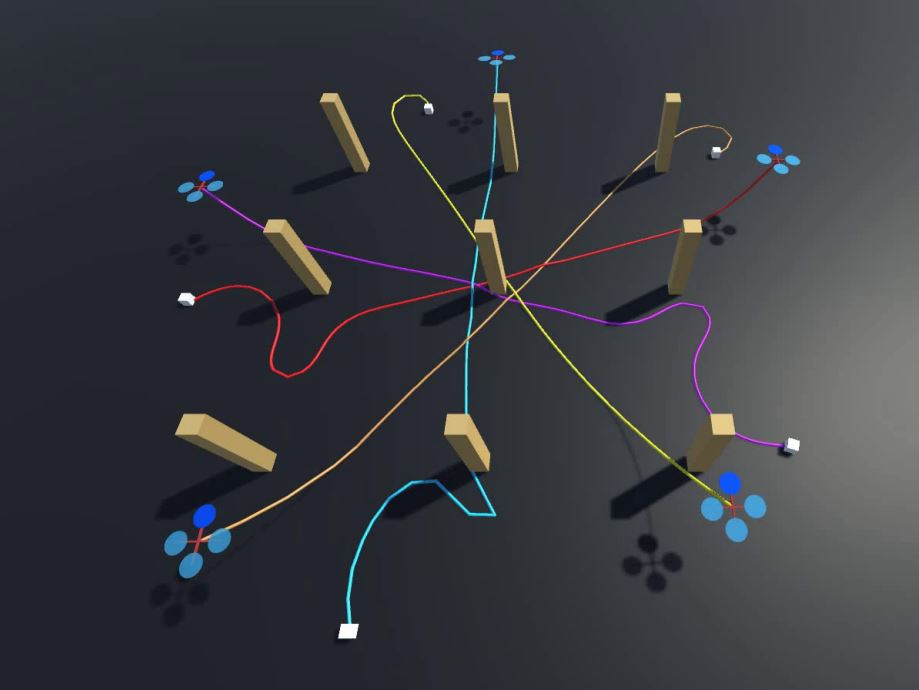 | Cao, Muqing; Cao, Kun; Yuan, Shenghai; Nguyen, Thien-Minh; Xie, Lihua: NEPTUNE: Non-Entangling Planning for Multiple Tethered Unmanned Vehicles. In: arxiv:2212.01536, 2022. @article{Cao2022,
title = {NEPTUNE: Non-Entangling Planning for Multiple Tethered Unmanned Vehicles},
author = {Cao, Muqing and Cao, Kun and Yuan, Shenghai and Nguyen, Thien-Minh and Xie, Lihua},
url = {https://arxiv.org/abs/2212.01536
https://arxiv.org/pdf/2212.01536},
year = {2022},
date = {2022-04-01},
journal = {arxiv:2212.01536},
keywords = {External},
pubstate = {published},
tppubtype = {article}
}
|
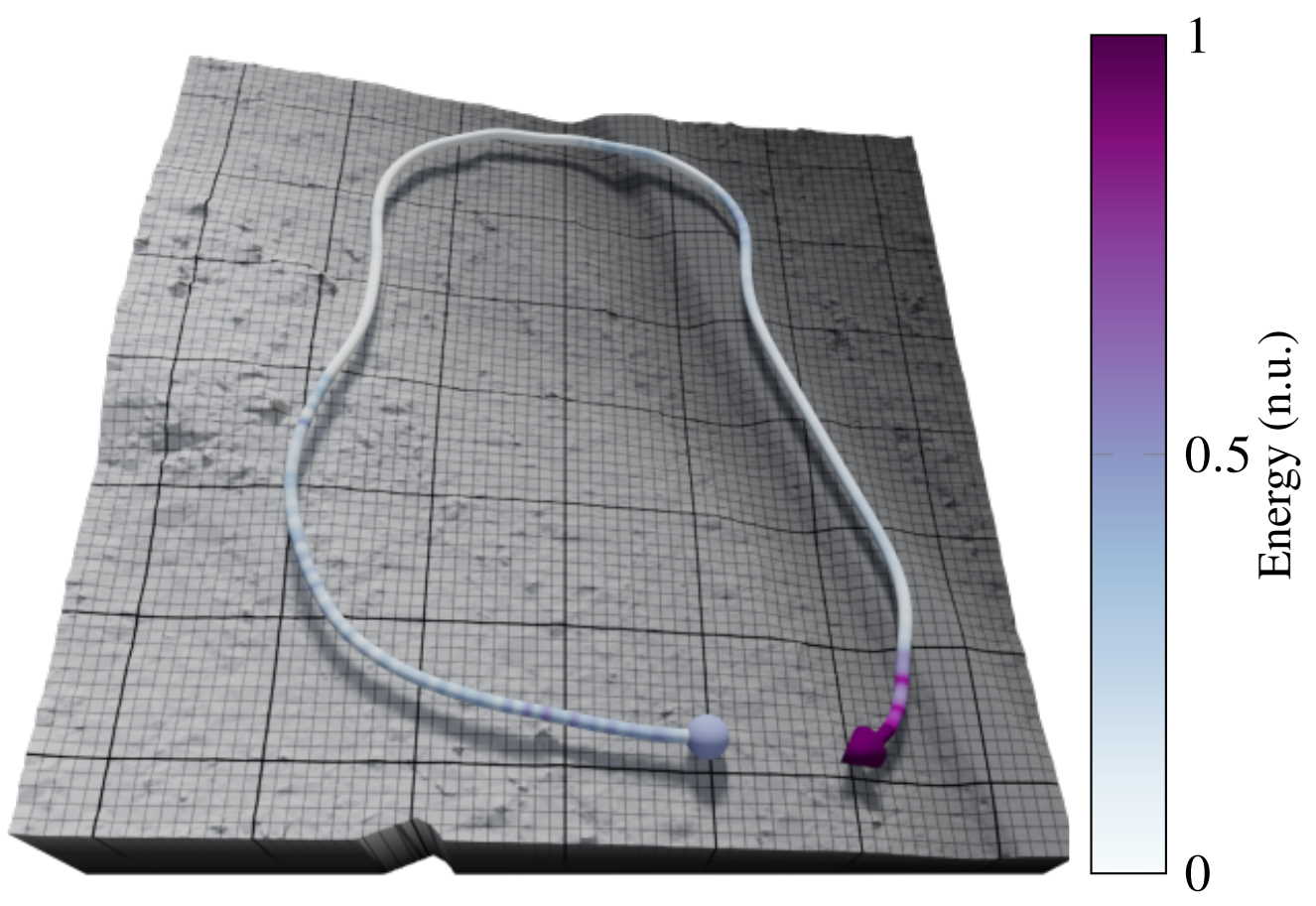 | Wallin, E.; Wiberg, V.; Vesterlund, F.; Holmgren, J.; Persson, H.; Servin, M.: Learning multiobjective rough terrain traversability. In: Journal of Terramechanics, vol. 102, pp. 17-26, 2022. @article{wallin2022,
title = {Learning multiobjective rough terrain traversability},
author = {Wallin, E. and Wiberg, V. and Vesterlund, F. and Holmgren, J. and Persson, H. and Servin, M.},
url = {https://authors.elsevier.com/sd/article/S0022489822000313
https://www.sciencedirect.com/science/article/pii/S0022489822000313/pdfft?md5=5dda8fdd1e395ea0e205c16deda5aed4&pid=1-s2.0-S0022489822000313-main.pdf
https://arxiv.org/pdf/2203.16354.pdf},
doi = {10.1016/j.jterra.2022.04.002 },
year = {2022},
date = {2022-04-01},
journal = {Journal of Terramechanics},
volume = {102},
pages = {17-26},
abstract = {We present a method that uses high-resolution topography data of rough terrain and ground vehicle simulation to predict traversability. Traversability is expressed as three independent measures: the ability to traverse the terrain at a target speed, energy consumption, and acceleration. The measures are continuous and reflect different objectives for planning that go beyond binary classification. A deep neural network is trained to predict the traversability measures from the local heightmap and target speed. To produce training data, we use an articulated vehicle with wheeled bogie suspensions and procedurally generated terrains. We evaluate the model on laser-scanned forest terrains, previously unseen by the model. The model predicts traversability with an accuracy of 90%. Predictions rely on features from the high-dimensional terrain data that surpass local roughness and slope relative to the heading. Correlations show that the three traversability measures are complementary to each other. With an inference speed 3000 times faster than the ground truth simulation and trivially parallelizable, the model is well suited for traversability analysis and optimal route planning over large areas.},
keywords = {External},
pubstate = {published},
tppubtype = {article}
}
We present a method that uses high-resolution topography data of rough terrain and ground vehicle simulation to predict traversability. Traversability is expressed as three independent measures: the ability to traverse the terrain at a target speed, energy consumption, and acceleration. The measures are continuous and reflect different objectives for planning that go beyond binary classification. A deep neural network is trained to predict the traversability measures from the local heightmap and target speed. To produce training data, we use an articulated vehicle with wheeled bogie suspensions and procedurally generated terrains. We evaluate the model on laser-scanned forest terrains, previously unseen by the model. The model predicts traversability with an accuracy of 90%. Predictions rely on features from the high-dimensional terrain data that surpass local roughness and slope relative to the heading. Correlations show that the three traversability measures are complementary to each other. With an inference speed 3000 times faster than the ground truth simulation and trivially parallelizable, the model is well suited for traversability analysis and optimal route planning over large areas. |
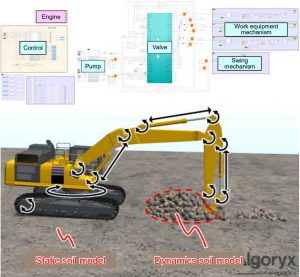 | Shintani, T.; Saito, Y.; Kiritani, Y.; Ozawa, S.; Obayashi, K.: Applying Model-based Development to Performance Development of Hydraulic Excavators Using 1DCAE. Komatsu Technical Report no. Vol.67 No.174, 2022. @techreport{Shintani2022,
title = {Applying Model-based Development to Performance Development of Hydraulic Excavators Using 1DCAE},
author = {Shintani, T. and Saito, Y. and Kiritani, Y. and Ozawa, S. and Obayashi, K.},
url = {https://www.komatsu.jp/en/-/media/home/aboutus/innovation/technology/techreport/2021/en/174e01.pdf},
year = {2022},
date = {2022-03-31},
number = {Vol.67 No.174},
institution = {Komatsu Technical Report},
abstract = {The requirements for construction machinery are becoming more sophisticated year by year, and the systems are becoming larger and more complex. Komatsu is aiming for efficient development even in large and complicated systems by applying model-based development to vehicle performance development. This paper reports an application example of using 1DCAE for the performance development of a hydraulic excavator, and also introduces examples of further utilization of 1DCAE such as a simulator.},
keywords = {External},
pubstate = {published},
tppubtype = {techreport}
}
The requirements for construction machinery are becoming more sophisticated year by year, and the systems are becoming larger and more complex. Komatsu is aiming for efficient development even in large and complicated systems by applying model-based development to vehicle performance development. This paper reports an application example of using 1DCAE for the performance development of a hydraulic excavator, and also introduces examples of further utilization of 1DCAE such as a simulator. |
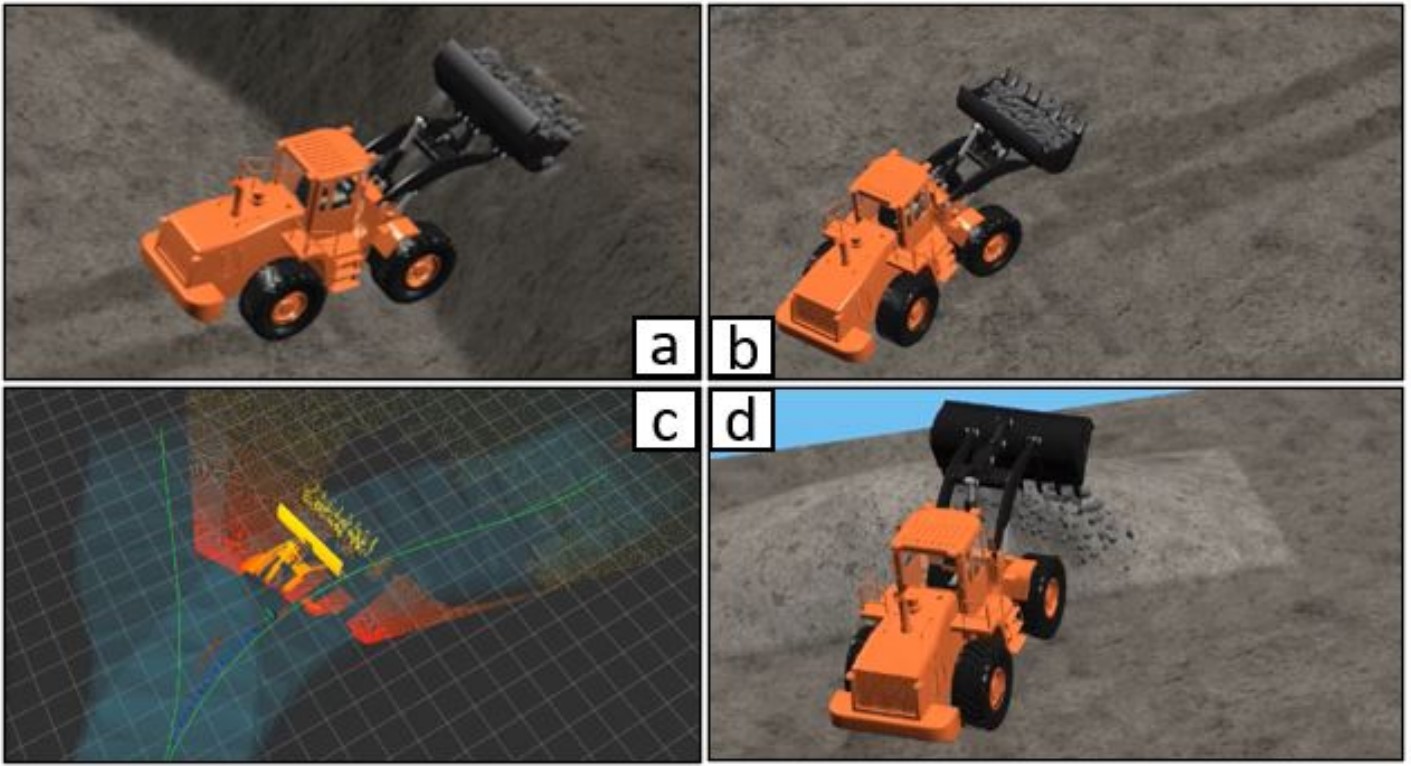 | Song, Ruitao; Ye, Zhixian; Wang, Liyang; He, Tianyi; Zhang, Liangjun: Autonomous Wheel Loader Trajectory Tracking Control Using LPV-MPC. In: arxiv:2203.08944, 2022. @article{Song2022,
title = {Autonomous Wheel Loader Trajectory Tracking Control Using LPV-MPC},
author = {Song, Ruitao and Ye, Zhixian and Wang, Liyang and He, Tianyi and Zhang, Liangjun},
url = {https://arxiv.org/abs/2203.08944
https://youtu.be/QbNfS_wZKKA},
doi = {https://doi.org/10.48550/arxiv.2203.08944},
year = {2022},
date = {2022-03-30},
journal = {arxiv:2203.08944},
abstract = {In this paper, we present a systematic approach for high-performance and efficient trajectory tracking control of autonomous wheel loaders. With the nonlinear dynamic model of a wheel loader, nonlinear model predictive control (MPC) is used in offline trajectory planning to obtain a high-performance state-control trajectory while satisfying the state and control constraints. In tracking control, the nonlinear model is embedded into a Linear Parameter Varying (LPV) model and the LPV-MPC strategy is used to achieve fast online computation and good tracking performance. To demonstrate the effectiveness and the advantages of the LPV-MPC, we test and compare three model predictive control strategies in the high-fidelity simulation environment. With the planned trajectory, three tracking control strategies LPV-MPC, nonlinear MPC, and LTI-MPC are simulated and compared in the perspectives of computational burden and tracking performance. The LPV-MPC can achieve better performance than conventional LTI-MPC because more accurate nominal system dynamics are captured in the LPV model. In addition, LPV-MPC achieves slightly worse tracking performance but tremendously improved computational efficiency than nonlinear MPC. A video with loading cycles completed by our autonomous wheel loader in the simulation environment can be found here: this https URL.},
keywords = {External},
pubstate = {published},
tppubtype = {article}
}
In this paper, we present a systematic approach for high-performance and efficient trajectory tracking control of autonomous wheel loaders. With the nonlinear dynamic model of a wheel loader, nonlinear model predictive control (MPC) is used in offline trajectory planning to obtain a high-performance state-control trajectory while satisfying the state and control constraints. In tracking control, the nonlinear model is embedded into a Linear Parameter Varying (LPV) model and the LPV-MPC strategy is used to achieve fast online computation and good tracking performance. To demonstrate the effectiveness and the advantages of the LPV-MPC, we test and compare three model predictive control strategies in the high-fidelity simulation environment. With the planned trajectory, three tracking control strategies LPV-MPC, nonlinear MPC, and LTI-MPC are simulated and compared in the perspectives of computational burden and tracking performance. The LPV-MPC can achieve better performance than conventional LTI-MPC because more accurate nominal system dynamics are captured in the LPV model. In addition, LPV-MPC achieves slightly worse tracking performance but tremendously improved computational efficiency than nonlinear MPC. A video with loading cycles completed by our autonomous wheel loader in the simulation environment can be found here: this https URL. |
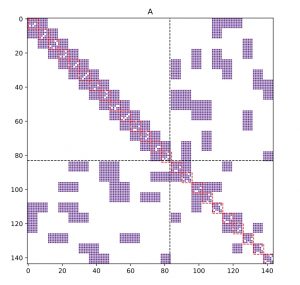 | Despaigne, Henrik: Fibre-based preconditioner for granular matter simulation. Department of Computing Science, Umeå University, 2022. @mastersthesis{Despaigne2022,
title = {Fibre-based preconditioner for granular matter simulation},
author = {Henrik Despaigne},
url = {https://www.diva-portal.org/smash/record.jsf?pid=diva2:1638655
https://www.diva-portal.org/smash/get/diva2:1638655/FULLTEXT01.pdf},
year = {2022},
date = {2022-02-18},
school = {Department of Computing Science, Umeå University},
abstract = {Simulating granular media at large scales is hard to do because of ill-conditioning of the associated linear systems and the ineffectiveness of available iterative methods. One common way to improve iterative methods is to use a preconditioner which involves finding a good approximation of a linear system A. A good preconditioner will improve the condition number of A. If a linear system has a set of large eigenvalues of comparable magnitude, and the rest of the eigenvalues are small, so that the gap between the set of large eigenvalues and the small ones is large, the ill-conditioning caused by the small eigenvalues will not appear in the early iterations. We investigate a new fibre-based preconditioner that involves finding chains of contacting particles along the particles of a granular medium and reordering the system, which leads to a diagonal preconditioner. We show its effects on the relative residual and error of the velocity on linear systems where the ill-conditioning is caused by a big gap between a set of large eigenvalues and small eigenvalues for three differentiterative methods: Uzawa, the Conjugate Residual (CR) and the Minimum ResidualMethod (MINRES).},
keywords = {Algoryx},
pubstate = {published},
tppubtype = {mastersthesis}
}
Simulating granular media at large scales is hard to do because of ill-conditioning of the associated linear systems and the ineffectiveness of available iterative methods. One common way to improve iterative methods is to use a preconditioner which involves finding a good approximation of a linear system A. A good preconditioner will improve the condition number of A. If a linear system has a set of large eigenvalues of comparable magnitude, and the rest of the eigenvalues are small, so that the gap between the set of large eigenvalues and the small ones is large, the ill-conditioning caused by the small eigenvalues will not appear in the early iterations. We investigate a new fibre-based preconditioner that involves finding chains of contacting particles along the particles of a granular medium and reordering the system, which leads to a diagonal preconditioner. We show its effects on the relative residual and error of the velocity on linear systems where the ill-conditioning is caused by a big gap between a set of large eigenvalues and small eigenvalues for three differentiterative methods: Uzawa, the Conjugate Residual (CR) and the Minimum ResidualMethod (MINRES). |
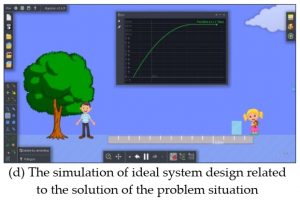 | Ürek, Handan; Çoramik, Mustafa: A Suggestion and Evaluation of a STEM Activity about Friction Coefficient for Pre-Service Science Teachers. In: Journal of Computer and Education Research, vol. 10, no. 19, pp. 202 - 235, 2022. @article{Urek2022,
title = {A Suggestion and Evaluation of a STEM Activity about Friction Coefficient for Pre-Service Science Teachers},
author = {Handan Ürek and Mustafa Çoramik},
url = {https://dergipark.org.tr/en/pub/jcer/issue/69325/1063301
https://dergipark.org.tr/en/download/article-file/2214073},
doi = {10.18009/jcer.1063301},
year = {2022},
date = {2022-01-02},
journal = {Journal of Computer and Education Research},
volume = {10},
number = {19},
pages = {202 - 235},
abstract = {In this study, the process of developing and evaluating a STEM activity which can be implemented during Science Teaching Laboratory Practice course in accordance with the 5E Model related to the concept of friction coefficient was addressed. The implementation of the activity was conducted in the form of a case study with the participation of 16 third year pre-service science teachers. Student journal forms and worksheets were utilized for the evaluation of the activity. As a result, it was determined that planned activity could be successfully applied to the pre-service teachers during the weekly course hours of Science Teaching Laboratory Practice course. In addition, positive feedbacks were obtained from pre-service teachers’ evaluation for the activity. It is believed that such studies, which establish connections between science and different disciplines, can contribute to the training of qualified science teachers and that such studies should be given more space in science teacher education.
},
keywords = {External},
pubstate = {published},
tppubtype = {article}
}
In this study, the process of developing and evaluating a STEM activity which can be implemented during Science Teaching Laboratory Practice course in accordance with the 5E Model related to the concept of friction coefficient was addressed. The implementation of the activity was conducted in the form of a case study with the participation of 16 third year pre-service science teachers. Student journal forms and worksheets were utilized for the evaluation of the activity. As a result, it was determined that planned activity could be successfully applied to the pre-service teachers during the weekly course hours of Science Teaching Laboratory Practice course. In addition, positive feedbacks were obtained from pre-service teachers’ evaluation for the activity. It is believed that such studies, which establish connections between science and different disciplines, can contribute to the training of qualified science teachers and that such studies should be given more space in science teacher education.
|
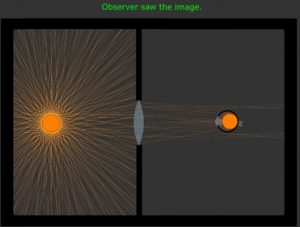 | Mustafa, Erdoğan Özdemir Coramik: Development of a virtual teaching environment with Algodoo: eye and cactus type light source models. In: Physics Education, vol. 57, no. 4, pp. 045022, 2022. @article{Özdemir2022,
title = {Development of a virtual teaching environment with Algodoo: eye and cactus type light source models},
author = {Erdoğan Özdemir
Coramik Mustafa},
url = {https://iopscience.iop.org/article/10.1088/1361-6552/ac60b0
https://iopscience.iop.org/article/10.1088/1361-6552/ac60b0/pdf?casa_token=V8xrPx7vhDAAAAAA:rPLZ6ro88reQ4VsHcF3r17FcMFZS5zgzLEr7VJMegEl3W8ni7Xy9hDyRQKmWdtQBQqQDDVQR},
doi = {10.1088/1361-6552/ac60b0},
year = {2022},
date = {2022-01-01},
journal = {Physics Education},
volume = {57},
number = {4},
pages = {045022},
abstract = {It is often necessary to enrich the teaching environment in order for students to learn optics in depth and to interpret the real optical situations with the information they have learned. In this study, a virtual teaching environment was developed using by Algodoo, a 2D simulation software. An eye model was created in order to explain the formation of the image in the eye in the teaching environment. Also, a cactus type light source model has also been developed to demonstrate that the image can be created in optics by using rays other than special rays. In order to show the success of the virtual teaching environment in modelling, previously known situations that the students had difficulty in understanding were simulated. Finally, some suggestions were made about the use of the virtual teaching environment. },
keywords = {External},
pubstate = {published},
tppubtype = {article}
}
It is often necessary to enrich the teaching environment in order for students to learn optics in depth and to interpret the real optical situations with the information they have learned. In this study, a virtual teaching environment was developed using by Algodoo, a 2D simulation software. An eye model was created in order to explain the formation of the image in the eye in the teaching environment. Also, a cactus type light source model has also been developed to demonstrate that the image can be created in optics by using rays other than special rays. In order to show the success of the virtual teaching environment in modelling, previously known situations that the students had difficulty in understanding were simulated. Finally, some suggestions were made about the use of the virtual teaching environment. |
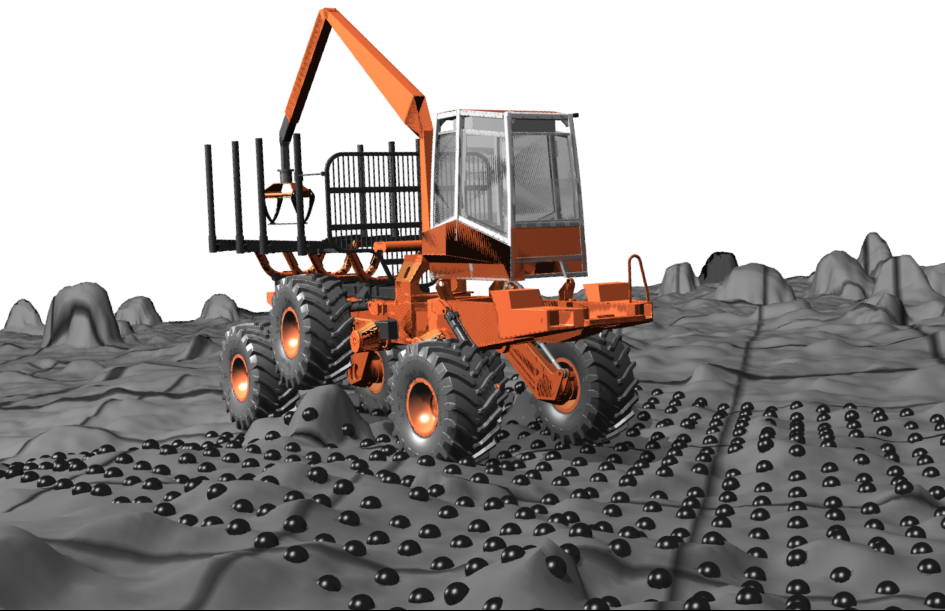 | Wiberg, Viktor; Wallin, Erik; Servin, Martin; Nordfjell, Tomas: Control of rough terrain vehicles using deep reinforcement learning. In: IEEE Robotics and Automation Letters, vol. 7, no. 1, pp. 390-397, 2022. @article{wiberg2021b,
title = {Control of rough terrain vehicles using deep reinforcement learning},
author = {Viktor Wiberg and Erik Wallin and Martin Servin and Tomas Nordfjell},
url = {https://arxiv.org/abs/2107.01867
http://umit.cs.umu.se/control_terrain/},
doi = {https://ieeexplore.ieee.org/document/9611016},
year = {2022},
date = {2022-01-01},
journal = {IEEE Robotics and Automation Letters},
volume = {7},
number = {1},
pages = {390-397},
abstract = {We explore the potential to control terrain vehicles using deep reinforcement in scenarios where human operators and traditional control methods are inadequate. This letter presents a controller that perceives, plans, and successfully controls a 16-tonne forestry vehicle with two frame articulation joints, six wheels, and their actively articulated suspensions to traverse rough terrain. The carefully shaped reward signal promotes safe, environmental, and efficient driving, which leads to the emergence of unprecedented driving skills. We test learned skills in a virtual environment, including terrains reconstructed from high-density laser scans of forest sites. The controller displays the ability to handle obstructing obstacles, slopes up to 27°, and a variety of natural terrains, all with limited wheel slip, smooth, and upright traversal with intelligent use of the active suspensions. The results confirm that deep reinforcement learning has the potential to enhance control of vehicles with complex dynamics and high-dimensional observation data compared to human operators or traditional control methods, especially in rough terrain.
},
howpublished = { arXiv:2107.01867},
keywords = {Algoryx},
pubstate = {published},
tppubtype = {article}
}
We explore the potential to control terrain vehicles using deep reinforcement in scenarios where human operators and traditional control methods are inadequate. This letter presents a controller that perceives, plans, and successfully controls a 16-tonne forestry vehicle with two frame articulation joints, six wheels, and their actively articulated suspensions to traverse rough terrain. The carefully shaped reward signal promotes safe, environmental, and efficient driving, which leads to the emergence of unprecedented driving skills. We test learned skills in a virtual environment, including terrains reconstructed from high-density laser scans of forest sites. The controller displays the ability to handle obstructing obstacles, slopes up to 27°, and a variety of natural terrains, all with limited wheel slip, smooth, and upright traversal with intelligent use of the active suspensions. The results confirm that deep reinforcement learning has the potential to enhance control of vehicles with complex dynamics and high-dimensional observation data compared to human operators or traditional control methods, especially in rough terrain.
|
2021
|
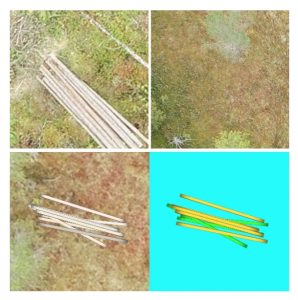 | Andersson, Jennifer; Servin, Martin: Predicting Gripability Heatmaps using Conditional GANs. Department of Physics. Umeå University Technical report, no. diva2:1622970, 2021. @techreport{Andersson2021b,
title = {Predicting Gripability Heatmaps using Conditional GANs},
author = {Jennifer Andersson and Martin Servin},
url = {https://www.diva-portal.org/smash/record.jsf?pid=diva2:1622970
https://www.diva-portal.org/smash/get/diva2:1622970/FULLTEXT01.pdf},
year = {2021},
date = {2021-12-15},
number = {diva2:1622970},
institution = {Department of Physics. Umeå University},
abstract = {The feasibility of using conditional GANs (Generative Adversarial Networks) to predict gripability in log piles is investigated. This is done by posing gripability heatmap prediction from RGB-D data as an image-to-image translation problem. Conditional GANs have previously achieved impressive results on several image-to-image translation tasks predicting physical properties and adding details not present in the input images. Here, piles of logs modelled as sticks or rods are generated in simulation, and groundtruth gripability maps are created using a simple algorithm streamlining the datacollection process. A modified SSIM (Structural Similarity Index) is used to evaluate the quality of the gripability heatmap predictions. The results indicate promising model performance on several different datasets and heatmap designs, including using base plane textures from a real forest production site to add realistic noise in the RGB data. Including a depth channel in the input data is shown to increase performance compared to using pure RGB data. The implementation is based on the general Pix2Pix network developed by Isola et al. in 2017. However, there is potential to increase performance and model generalization, and the adoption of more advanced loss functions and network architectures are suggested. Next steps include using terrains reconstructed from highdensity laser scans in physics-based simulation for data generation. A more in-depth discussion regarding the level of sophistication required in the gripability heatmaps should also be carried out, along with discussions regarding other specifications that will be required for future deployment. This will enable derivation of a tailored gripability metric for ground-truth heatmap generation, and method evaluation on less ideal data.},
type = {Technical report},
keywords = {External},
pubstate = {published},
tppubtype = {techreport}
}
The feasibility of using conditional GANs (Generative Adversarial Networks) to predict gripability in log piles is investigated. This is done by posing gripability heatmap prediction from RGB-D data as an image-to-image translation problem. Conditional GANs have previously achieved impressive results on several image-to-image translation tasks predicting physical properties and adding details not present in the input images. Here, piles of logs modelled as sticks or rods are generated in simulation, and groundtruth gripability maps are created using a simple algorithm streamlining the datacollection process. A modified SSIM (Structural Similarity Index) is used to evaluate the quality of the gripability heatmap predictions. The results indicate promising model performance on several different datasets and heatmap designs, including using base plane textures from a real forest production site to add realistic noise in the RGB data. Including a depth channel in the input data is shown to increase performance compared to using pure RGB data. The implementation is based on the general Pix2Pix network developed by Isola et al. in 2017. However, there is potential to increase performance and model generalization, and the adoption of more advanced loss functions and network architectures are suggested. Next steps include using terrains reconstructed from highdensity laser scans in physics-based simulation for data generation. A more in-depth discussion regarding the level of sophistication required in the gripability heatmaps should also be carried out, along with discussions regarding other specifications that will be required for future deployment. This will enable derivation of a tailored gripability metric for ground-truth heatmap generation, and method evaluation on less ideal data. |
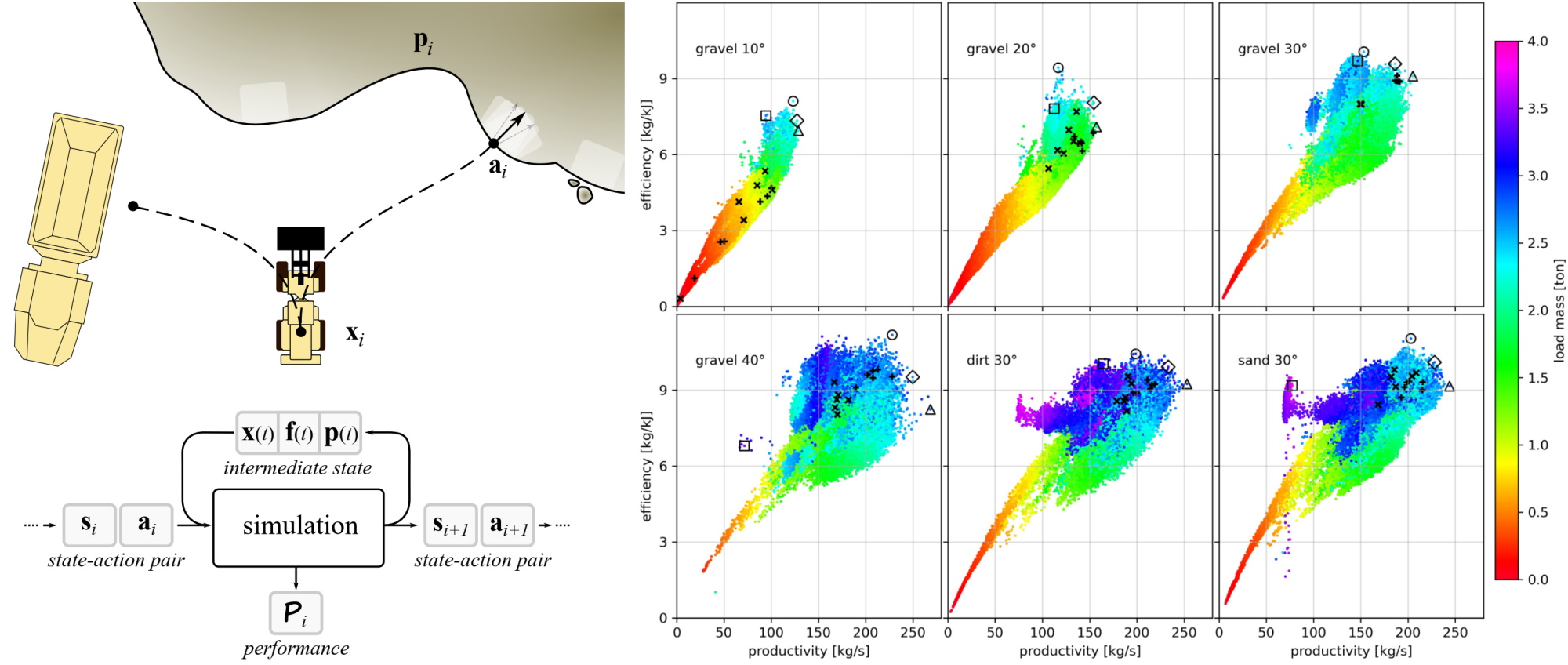 | Aoshima, Koji; Servin, Martin; Wadbro, Eddie: Simulation-Based Optimization of High-Performance Wheel Loading. Proceedings of the 38th International Symposium on Automation and Robotics in Construction (ISARC), International Association for Automation and Robotics in Construction (IAARC), 2021, ISBN: 978-952-69524-1-3. @conference{Aoshima2021,
title = {Simulation-Based Optimization of High-Performance Wheel Loading},
author = {Koji Aoshima and Martin Servin and Eddie Wadbro},
editor = {Feng, Chen and Linner, Thomas and Brilakis, Ioannis and Castro, Daniel and Chen, Po-Han and Cho, Yong and Du, Jing and Ergan, Semiha and Garcia de Soto, Borja and Gaparík, Jozef and Habbal, Firas and Hammad, Amin and Iturralde, Kepa and Bock, Thomas and Kwon, Soonwook and Lafhaj, Zoubeir and Li, Nan and Liang, Ci-Jyun and Mantha, Bharadwaj and Ng, Ming Shan and Hall, Daniel and Pan, Mi and Pan, Wei and Rahimian, Farzad and Raphael, Benny and Sattineni, Anoop and Schlette, Christian and Shabtai, Isaac and Shen, Xuesong and Tang, Pingbo and Teizer, Jochen and Turkan, Yelda and Valero, Enrique and Zhu, Zhenhua},
url = {https://www.iaarc.org/publications/2021_proceedings_of_the_38th_isarc/simulation_based_optimization_of_high_performance_wheel_loading.html
https://arxiv.org/abs/2107.14615
http://umit.cs.umu.se/hp_loading/},
doi = {10.22260/ISARC2021/009310.22260/ISARC2021/0093},
isbn = {978-952-69524-1-3},
year = {2021},
date = {2021-08-02},
booktitle = {Proceedings of the 38th International Symposium on Automation and Robotics in Construction (ISARC)},
pages = {688-695},
publisher = {International Association for Automation and Robotics in Construction (IAARC)},
abstract = {Having smart and autonomous earthmoving in mind, we explore high-performance wheel loading in a simulated environment. This paper introduces a wheel loader simulator that combines contacting 3D multibody dynamics with a hybrid continuum-particle terrain model, supporting realistic digging forces and soil displacements at real-time performance. A total of 270,000 simulations are run with different loading actions, pile slopes, and soil to analyze how they affect the loading performance. The results suggest that the preferred digging actions should preserve and exploit a steep pile slope. High digging speed favors high productivity, while energy-efficient loading requires a lower dig speed.},
howpublished = {38th International Symposium on Automation and Robotics in Construction (ISARC), Dubai, UAE (2021). arXiv:2107.14615 },
keywords = {Algoryx, External},
pubstate = {published},
tppubtype = {conference}
}
Having smart and autonomous earthmoving in mind, we explore high-performance wheel loading in a simulated environment. This paper introduces a wheel loader simulator that combines contacting 3D multibody dynamics with a hybrid continuum-particle terrain model, supporting realistic digging forces and soil displacements at real-time performance. A total of 270,000 simulations are run with different loading actions, pile slopes, and soil to analyze how they affect the loading performance. The results suggest that the preferred digging actions should preserve and exploit a steep pile slope. High digging speed favors high productivity, while energy-efficient loading requires a lower dig speed. |
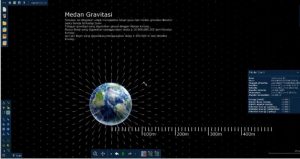 | Susanti, D; Yaqiina, MH; Maulana, S: Newton’s Gravity Interactive Simulation to Improve 10th-Grade Students’ Learning Outcome. Journal of Physics: Conference Series, vol. 2377, no. 1, IOP Publishing, 2021. @conference{susanti2022newton,
title = {Newton’s Gravity Interactive Simulation to Improve 10th-Grade Students’ Learning Outcome},
author = {Susanti, D and Yaqiina, MH and Maulana, S},
url = {https://iopscience.iop.org/article/10.1088/1742-6596/2377/1/012091/meta},
year = {2021},
date = {2021-06-01},
booktitle = {Journal of Physics: Conference Series},
volume = {2377},
number = {1},
pages = {012091},
publisher = {IOP Publishing},
keywords = {External},
pubstate = {published},
tppubtype = {conference}
}
|
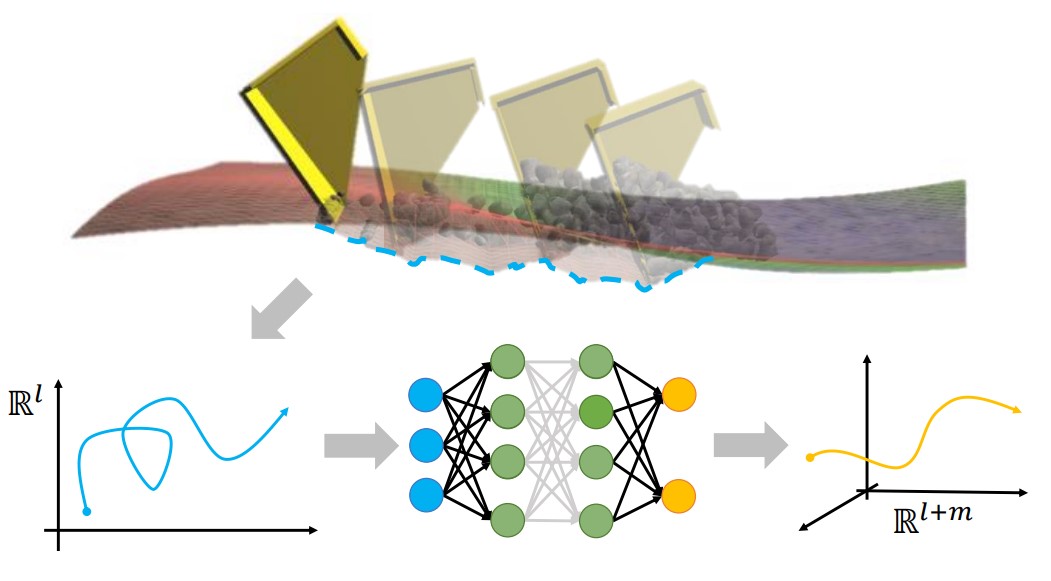 | Selby, Nicholas S; Asada, Harry H: Learning of Causal Observable Functions for Koopman-DFL Lifting Linearization of Nonlinear Controlled Systems and Its Application to Excavation Automation. In: IEEE Robotics and Automation Letters, vol. 6, no. 4, pp. 6297 - 6304, 2021. @article{selby2021learning,
title = {Learning of Causal Observable Functions for Koopman-DFL Lifting Linearization of Nonlinear Controlled Systems and Its Application to Excavation Automation},
author = {Nicholas S Selby and Harry H Asada},
url = {https://arxiv.org/abs/2104.02004
https://arxiv.org/pdf/2104.02004
},
doi = {https://doi.org/10.1109/LRA.2021.3092256},
year = {2021},
date = {2021-06-01},
journal = {IEEE Robotics and Automation Letters},
volume = {6},
number = {4},
pages = {6297 - 6304},
abstract = {Effective and causal observable functions for low-order lifting linearization of nonlinear controlled systems are learned from data by using neural networks. While Koopman operator theory allows us to represent a nonlinear system as a linear system in an infinite-dimensional space of observables, exact linearization is guaranteed only for autonomous systems with no input, and finding effective observable functions for approximation with a low-order linear system remains an open question. Dual-Faceted Linearization uses a set of effective observables for low-order lifting linearization, but the method requires knowledge of the physical structure of the nonlinear system. Here, a data-driven method is presented for generating a set of nonlinear observable functions that can accurately approximate a nonlinear control system to a low-order linear control system. A caveat in using data of measured variables as observables is that the measured variables may contain input to the system, which incurs a causality contradiction when lifting the system, i.e., taking derivatives of the observables. The current work presents a method for eliminating such anti-causal components of the observables and lifting the system using only causal observables. The method is applied to excavation automation, a complex nonlinear dynamical system, to obtain a low-order lifted linear model for control design.},
keywords = {External},
pubstate = {published},
tppubtype = {article}
}
Effective and causal observable functions for low-order lifting linearization of nonlinear controlled systems are learned from data by using neural networks. While Koopman operator theory allows us to represent a nonlinear system as a linear system in an infinite-dimensional space of observables, exact linearization is guaranteed only for autonomous systems with no input, and finding effective observable functions for approximation with a low-order linear system remains an open question. Dual-Faceted Linearization uses a set of effective observables for low-order lifting linearization, but the method requires knowledge of the physical structure of the nonlinear system. Here, a data-driven method is presented for generating a set of nonlinear observable functions that can accurately approximate a nonlinear control system to a low-order linear control system. A caveat in using data of measured variables as observables is that the measured variables may contain input to the system, which incurs a causality contradiction when lifting the system, i.e., taking derivatives of the observables. The current work presents a method for eliminating such anti-causal components of the observables and lifting the system using only causal observables. The method is applied to excavation automation, a complex nonlinear dynamical system, to obtain a low-order lifted linear model for control design. |
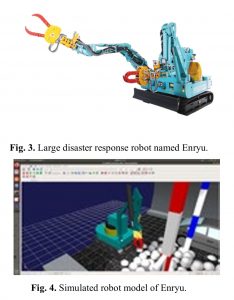 | Shima, Daichi; Furukawa, Tomoyuki; Aoba, Ryuma; Ohashi, Ayato; Tsuruno, Kota; Naruse, Keitaro: Team Activity of Robot Competition of Simulated Robot in World Robot Summit 2020. In: SHS Web of Conferences, pp. 04016, EDP Sciences 2021, ISSN: 2261-2424. @inproceedings{shima2021team,
title = {Team Activity of Robot Competition of Simulated Robot in World Robot Summit 2020},
author = {Daichi Shima and Tomoyuki Furukawa and Ryuma Aoba and Ayato Ohashi and Kota Tsuruno and Keitaro Naruse},
url = {https://www.shs-conferences.org/articles/shsconf/abs/2021/13/shsconf_etltc2021_04016/shsconf_etltc2021_04016.html
https://www.shs-conferences.org/articles/shsconf/pdf/2021/13/shsconf_etltc2021_04016.pdf
},
issn = {2261-2424},
year = {2021},
date = {2021-05-08},
booktitle = {SHS Web of Conferences},
volume = {102},
pages = {04016},
organization = {EDP Sciences},
abstract = {World Robot Summit (WRS) has several robot competitions, and we will participate it in the infrastructure and disaster response category. Participating teams develop their robot system by teleoperation and/or autonomous operation and run it in a set of courses modelling and simplifying disaster responding situations. The authors will attend the challenge of the tunnel disaster response and recovery, in which we are requested to achieve an investigation and rescue scenario of a tunnel fire with simulated robots. As preparation, we develop simulated robot models and corresponding software as a team. In this article, we report out activity to the robot competition and student’s project-based learning by joining it.},
keywords = {External},
pubstate = {published},
tppubtype = {inproceedings}
}
World Robot Summit (WRS) has several robot competitions, and we will participate it in the infrastructure and disaster response category. Participating teams develop their robot system by teleoperation and/or autonomous operation and run it in a set of courses modelling and simplifying disaster responding situations. The authors will attend the challenge of the tunnel disaster response and recovery, in which we are requested to achieve an investigation and rescue scenario of a tunnel fire with simulated robots. As preparation, we develop simulated robot models and corresponding software as a team. In this article, we report out activity to the robot competition and student’s project-based learning by joining it. |
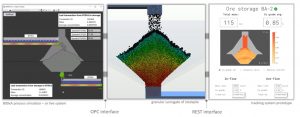 | Servin, Martin; Westerlund, Folke; Wallin, Erik: Digital twins with distributed particle simulation for mine-to-mill material tracking. In: Minerals, vol. 11, no. 5, pp. 524, 2021. @article{Servin2021b,
title = {Digital twins with distributed particle simulation for mine-to-mill material tracking},
author = {Martin Servin and Folke Westerlund and Erik Wallin},
url = {https://www.mdpi.com/2075-163X/11/5/524
https://arxiv.org/pdf/2104.09111.pdf},
doi = {https://doi.org/10.3390/min11050524},
year = {2021},
date = {2021-04-19},
journal = {Minerals},
volume = {11},
number = {5},
pages = {524},
abstract = {Systems for transport and processing of granular media are challenging to analyse, operate and optimise. In the mining and mineral processing industries these systems are chains of pro- cesses with complex interplay between the equipment, control, and the processed material. The material properties have natural variations that are usually only known at certain locations. Therefore, we explore a material-oriented approach to digital twins with a particle representa- tion of the granular media. In digital form, the material is treated as pseudo-particles, each representing a large collection of real particles of various sizes, shapes and, mineral properties. Movements and changes in the state of the material are determined by the combined data from control systems, sensors, vehicle telematics, and simulation models at locations where no real sensors can see. The particle-based representation enables material tracking along the chain of processes. Each digital particle can act as a carrier of observational data generated by the equipment as it interacts with the real material. This makes it possible to better learn material properties from process observations, and to predict the effect on downstream processes. We test the technique on a mining simulator and demonstrate analysis that can be performed using data from cross-system material tracking.
},
keywords = {Algoryx},
pubstate = {published},
tppubtype = {article}
}
Systems for transport and processing of granular media are challenging to analyse, operate and optimise. In the mining and mineral processing industries these systems are chains of pro- cesses with complex interplay between the equipment, control, and the processed material. The material properties have natural variations that are usually only known at certain locations. Therefore, we explore a material-oriented approach to digital twins with a particle representa- tion of the granular media. In digital form, the material is treated as pseudo-particles, each representing a large collection of real particles of various sizes, shapes and, mineral properties. Movements and changes in the state of the material are determined by the combined data from control systems, sensors, vehicle telematics, and simulation models at locations where no real sensors can see. The particle-based representation enables material tracking along the chain of processes. Each digital particle can act as a carrier of observational data generated by the equipment as it interacts with the real material. This makes it possible to better learn material properties from process observations, and to predict the effect on downstream processes. We test the technique on a mining simulator and demonstrate analysis that can be performed using data from cross-system material tracking.
|
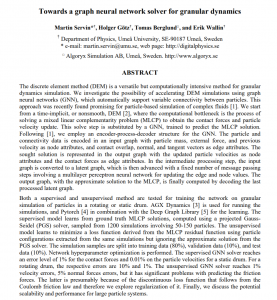 | Servin, Martin; Götz, Holger; Berglund, Tomas; Wallin, Erik: Towards a graph neural network solver for granular dynamics. VII International Conference on Particle-Based Methods (PARTICLES 2021), 2021. @conference{servintowards,
title = {Towards a graph neural network solver for granular dynamics},
author = {Martin Servin and Holger Götz and Tomas Berglund and Erik Wallin},
url = {https://www.researchgate.net/profile/Martin-Servin/publication/350089857_Towards_a_graph_neural_network_solver_for_granular_dynamics/links/6050749892851cd8ce445ca6/Towards-a-graph-neural-network-solver-for-granular-dynamics.pdf},
year = {2021},
date = {2021-03-01},
booktitle = {VII International Conference on Particle-Based Methods (PARTICLES 2021)},
abstract = {The discrete element method (DEM) is a versatile but computationally intensive method for granular dynamics simulation. We investigate the possibility of accelerating DEM simulations using graph neural networks (GNN), which automatically support variable connectivity between particles. This approach was recently found promising for particle-based simulation of complex fluids [1]. We start from a time-implicit, or nonsmooth, DEM [2], where the computational bottleneck is the process of solving a mixed linear complementarity problem (MLCP) to obtain the contact forces and particle velocity update. This solve step is substituted by a GNN, trained to predict the MLCP solution. Following [1], we employ an encoder-process-decoder structure for the GNN. The particle and connectivity data is encoded in an input graph with particle mass, external force, and previous velocity as node attributes, and contact overlap, normal, and tangent vectors as edge attributes. The sought solution is represented in the output graph with the updated particle velocities as node attributes and the contact forces as edge attributes. In the intermediate processing step, the input graph is converted to a latent graph, which is then advanced with a fixed number of message passing steps involving a multilayer perceptron neural network for updating the edge and node values. The output graph, with the approximate solution to the MLCP, is finally computed by decoding the last processed latent graph. Both a supervised and unsupervised method are tested for training the network on granular simulation of particles in a rotating or static drum. AGX Dynamics [3] is used for running the simulations, and Pytorch [4] in combination with the Deep Graph Library [5] for the learning. The supervised model learns from ground truth MLCP solutions, computed using a projected Gauss-Seidel (PGS) solver, sampled from 1200 simulations involving 50-150 particles. The unsupervised model learns to minimize a loss function derived from the MLCP residual function using particle configurations extracted from the same simulations but ignoring the approximate solution from the PGS solver. The simulation samples are split into training data (80%), validation data (10%), and test data (10%). Network hyperparameter optimization is performed. The supervised GNN solver reaches an error level of 1% for the contact forces and 0.01% on the particle velocities for a static drum. For a rotating drum, the respective errors are 10% and 1%. The unsupervised GNN solver reaches 1% velocity errors, 5% normal forces errors, but it has significant problems with predicting the friction forces. The latter is presumably because of the discontinuous loss function that follows from the Coulomb friction law and therefore we explore regularization of it. Finally, we discuss the potential scalability and performance for large particle systems.},
keywords = {Algoryx},
pubstate = {published},
tppubtype = {conference}
}
The discrete element method (DEM) is a versatile but computationally intensive method for granular dynamics simulation. We investigate the possibility of accelerating DEM simulations using graph neural networks (GNN), which automatically support variable connectivity between particles. This approach was recently found promising for particle-based simulation of complex fluids [1]. We start from a time-implicit, or nonsmooth, DEM [2], where the computational bottleneck is the process of solving a mixed linear complementarity problem (MLCP) to obtain the contact forces and particle velocity update. This solve step is substituted by a GNN, trained to predict the MLCP solution. Following [1], we employ an encoder-process-decoder structure for the GNN. The particle and connectivity data is encoded in an input graph with particle mass, external force, and previous velocity as node attributes, and contact overlap, normal, and tangent vectors as edge attributes. The sought solution is represented in the output graph with the updated particle velocities as node attributes and the contact forces as edge attributes. In the intermediate processing step, the input graph is converted to a latent graph, which is then advanced with a fixed number of message passing steps involving a multilayer perceptron neural network for updating the edge and node values. The output graph, with the approximate solution to the MLCP, is finally computed by decoding the last processed latent graph. Both a supervised and unsupervised method are tested for training the network on granular simulation of particles in a rotating or static drum. AGX Dynamics [3] is used for running the simulations, and Pytorch [4] in combination with the Deep Graph Library [5] for the learning. The supervised model learns from ground truth MLCP solutions, computed using a projected Gauss-Seidel (PGS) solver, sampled from 1200 simulations involving 50-150 particles. The unsupervised model learns to minimize a loss function derived from the MLCP residual function using particle configurations extracted from the same simulations but ignoring the approximate solution from the PGS solver. The simulation samples are split into training data (80%), validation data (10%), and test data (10%). Network hyperparameter optimization is performed. The supervised GNN solver reaches an error level of 1% for the contact forces and 0.01% on the particle velocities for a static drum. For a rotating drum, the respective errors are 10% and 1%. The unsupervised GNN solver reaches 1% velocity errors, 5% normal forces errors, but it has significant problems with predicting the friction forces. The latter is presumably because of the discontinuous loss function that follows from the Coulomb friction law and therefore we explore regularization of it. Finally, we discuss the potential scalability and performance for large particle systems. |
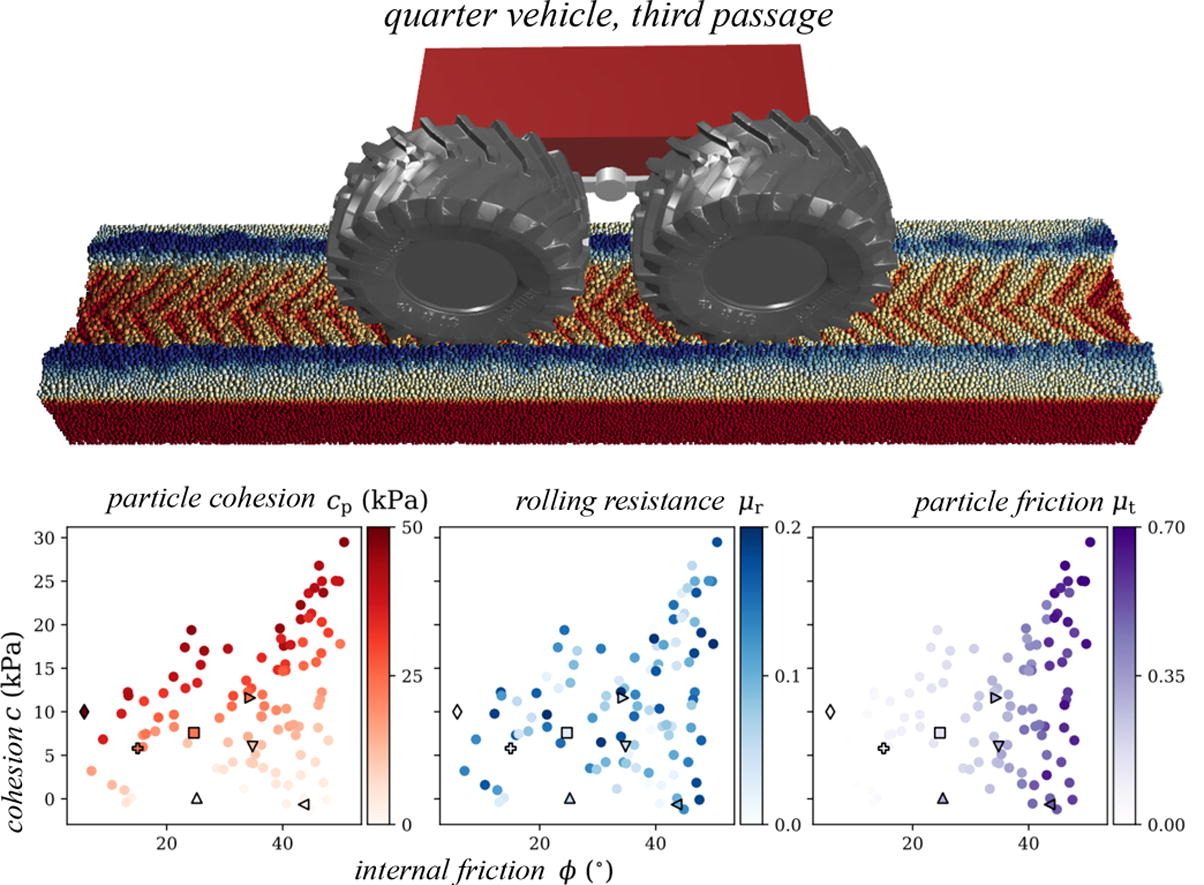 | Wiberg, Viktor; Servin, Martin; Nordfjell, Tomas: Discrete element modelling of large soil deformations under heavy vehicles. In: Journal of Terramechanics, vol. 93, pp. 11–21, 2021. @article{wiberg2021discrete,
title = {Discrete element modelling of large soil deformations under heavy vehicles},
author = {Viktor Wiberg and Martin Servin and Tomas Nordfjell},
url = {https://doi.org/10.1016/j.jterra.2020.10.002},
year = {2021},
date = {2021-01-01},
journal = {Journal of Terramechanics},
volume = {93},
pages = {11--21},
publisher = {Elsevier},
abstract = {This paper addresses the challenges of creating realistic models of soil for simulations of heavy vehicles on weak terrain. We modelled dense soils using the discrete element method with variable parameters for surface friction, normal cohesion, and rolling resistance. To find out what type of soils can be represented, we measured the internal friction and bulk cohesion of over 100 different virtual samples. To test the model, we simulated rut formation from a heavy vehicle with different loads and soil strengths. We conclude that the relevant space of dense frictional and frictional-cohesive soils can be represented and that the model is applicable for simulation of large deformations induced by heavy vehicles on weak terrain.},
keywords = {Algoryx},
pubstate = {published},
tppubtype = {article}
}
This paper addresses the challenges of creating realistic models of soil for simulations of heavy vehicles on weak terrain. We modelled dense soils using the discrete element method with variable parameters for surface friction, normal cohesion, and rolling resistance. To find out what type of soils can be represented, we measured the internal friction and bulk cohesion of over 100 different virtual samples. To test the model, we simulated rut formation from a heavy vehicle with different loads and soil strengths. We conclude that the relevant space of dense frictional and frictional-cohesive soils can be represented and that the model is applicable for simulation of large deformations induced by heavy vehicles on weak terrain. |
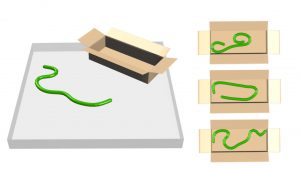 | Gieselmann, Robert; Pokorny, Florian T: Planning-Augmented Hierarchical Reinforcement Learning. In: IEEE Robotics and Automation Letters, vol. 6, no. 3, pp. 5097-5104, 2021. @article{Gieselmann2021,
title = {Planning-Augmented Hierarchical Reinforcement Learning},
author = {Robert Gieselmann and Florian T Pokorny},
doi = {10.1109/LRA.2021.3071062},
year = {2021},
date = {2021-01-01},
journal = {IEEE Robotics and Automation Letters},
volume = {6},
number = {3},
pages = {5097-5104},
abstract = {Abstract—Planning algorithms are powerful at solving longhorizon decision-making problems but require that environment dynamics are known. Model-free reinforcement learning has recently been merged with graph-based planning to increase the robustness of trained policies in state-space navigation problems. Recent ideas suggest to use planning in order to provide intermediate waypoints guiding the policy in long-horizon tasks. Yet, it is not always practical to describe a problem in the setting of state-to-state navigation. Often, the goal is defined by one or multiple disjoint sets of valid states or implicitly using an abstract task description. Building upon previous efforts, we introduce a novel algorithm called Planning-Augmented Hierarchical Reinforcement Learning (PAHRL) which translates the concept of hybrid planning/RL to such problems with implicitly defined goal. Using a hierarchical framework, we divide the original task, formulated as a Markov Decision Process (MDP), into a hierarchy of shorter horizon MDPs. Actor-critic agents are trained in parallel for each level of the hierarchy. During testing, a planner then determines useful subgoals on a state graph constructed at the bottom level of the hierarchy. The effectiveness of our approach is demonstrated for a set of continuous control problems in simulation including robot arm reaching tasks and the manipulation of a deformable object.},
keywords = {External},
pubstate = {published},
tppubtype = {article}
}
Abstract—Planning algorithms are powerful at solving longhorizon decision-making problems but require that environment dynamics are known. Model-free reinforcement learning has recently been merged with graph-based planning to increase the robustness of trained policies in state-space navigation problems. Recent ideas suggest to use planning in order to provide intermediate waypoints guiding the policy in long-horizon tasks. Yet, it is not always practical to describe a problem in the setting of state-to-state navigation. Often, the goal is defined by one or multiple disjoint sets of valid states or implicitly using an abstract task description. Building upon previous efforts, we introduce a novel algorithm called Planning-Augmented Hierarchical Reinforcement Learning (PAHRL) which translates the concept of hybrid planning/RL to such problems with implicitly defined goal. Using a hierarchical framework, we divide the original task, formulated as a Markov Decision Process (MDP), into a hierarchy of shorter horizon MDPs. Actor-critic agents are trained in parallel for each level of the hierarchy. During testing, a planner then determines useful subgoals on a state graph constructed at the bottom level of the hierarchy. The effectiveness of our approach is demonstrated for a set of continuous control problems in simulation including robot arm reaching tasks and the manipulation of a deformable object. |
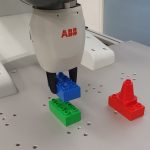 | Styrud, Jonathan; Iovino, Matteo; Norrlöf, Mikael; Björkman, Mårten; Smith, Christian: Combining Planning and Learning of Behavior Trees for Robotic Assembly. In: arXiv preprint arXiv:2103.09036, 2021. @article{styrud2021combining,
title = {Combining Planning and Learning of Behavior Trees for Robotic Assembly},
author = {Jonathan Styrud and Matteo Iovino and Mikael Norrlöf and Mårten Björkman and Christian Smith},
url = {https://arxiv.org/abs/2103.09036
https://arxiv.org/pdf/2103.09036
https://github.com/jstyrud/planning-and-learning
},
year = {2021},
date = {2021-01-01},
journal = {arXiv preprint arXiv:2103.09036},
abstract = {Industrial robots can solve very complex tasks in controlled environments, but modern applications require robots able to operate in unpredictable surroundings as well. An increasingly popular reactive policy architecture in robotics is Behavior Trees but as with other architectures, programming time still drives cost and limits flexibility. There are two main branches of algorithms to generate policies automatically, automated planning and machine learning, both with their own drawbacks. We propose a method for generating Behavior Trees using a Genetic Programming algorithm and combining the two branches by taking the result of an automated planner and inserting it into the population. Experimental results confirm that the proposed method of combining planning and learning performs well on a variety of robotic assembly problems and outperforms both of the base methods used separately. We also show that this type of high level learning of Behavior Trees can be transferred to a real system without further training.},
keywords = {External},
pubstate = {published},
tppubtype = {article}
}
Industrial robots can solve very complex tasks in controlled environments, but modern applications require robots able to operate in unpredictable surroundings as well. An increasingly popular reactive policy architecture in robotics is Behavior Trees but as with other architectures, programming time still drives cost and limits flexibility. There are two main branches of algorithms to generate policies automatically, automated planning and machine learning, both with their own drawbacks. We propose a method for generating Behavior Trees using a Genetic Programming algorithm and combining the two branches by taking the result of an automated planner and inserting it into the population. Experimental results confirm that the proposed method of combining planning and learning performs well on a variety of robotic assembly problems and outperforms both of the base methods used separately. We also show that this type of high level learning of Behavior Trees can be transferred to a real system without further training. |
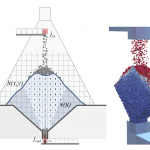 | Wallin, Erik; Servin, Martin: Data-driven model order reduction for granular media. In: Computational Particle Mechanics, pp. 1–14, 2021. @article{wallin2021data,
title = {Data-driven model order reduction for granular media},
author = {Erik Wallin and Martin Servin},
url = {http://umit.cs.umu.se/ddgranular/
https://doi.org/10.1007/s40571-020-00387-6
https://arxiv.org/pdf/2004.03349
https://arxiv.org/abs/2004.03349
https://youtu.be/YjwP9baTm-c?list=TLGGYuzbbp4IBtcxOTA0MjAyMQ},
year = {2021},
date = {2021-01-01},
journal = {Computational Particle Mechanics},
pages = {1--14},
publisher = {Springer},
abstract = {We investigate the use of reduced-order modelling to run discrete element simulations at higher speeds. Taking a data-driven approach, we run many offline simulations in advance and train a model to predict the velocity field from the mass distribution and system control signals. Rapid model inference of particle velocities replaces the intense process of computing contact forces and velocity updates. In coupled DEM and multibody system simulation the predictor model can be trained to output the interfacial reaction forces as well. An adaptive model order reduction technique is investigated, decomposing the media in domains of solid, liquid, and gaseous state. The model reduction is applied to solid and liquid domains where the particle motion is strongly correlated with the mean flow, while resolved DEM is used for gaseous domains. Using a ridge regression predictor, the performance is tested on simulations of a pile discharge and bulldozing. The measured accuracy is about 90% and 65%, respectively, and the speed-up range between 10 and 60},
keywords = {Algoryx},
pubstate = {published},
tppubtype = {article}
}
We investigate the use of reduced-order modelling to run discrete element simulations at higher speeds. Taking a data-driven approach, we run many offline simulations in advance and train a model to predict the velocity field from the mass distribution and system control signals. Rapid model inference of particle velocities replaces the intense process of computing contact forces and velocity updates. In coupled DEM and multibody system simulation the predictor model can be trained to output the interfacial reaction forces as well. An adaptive model order reduction technique is investigated, decomposing the media in domains of solid, liquid, and gaseous state. The model reduction is applied to solid and liquid domains where the particle motion is strongly correlated with the mean flow, while resolved DEM is used for gaseous domains. Using a ridge regression predictor, the performance is tested on simulations of a pile discharge and bulldozing. The measured accuracy is about 90% and 65%, respectively, and the speed-up range between 10 and 60 |
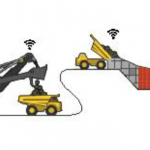 | Servin, Martin; Vesterlund, Folke; Wallin, Erik: Digital twins with embedded particle simulation. In: 14th World Congress in Computational Mechanics (WCCM) ECCOMAS Congress, Virtual, January 11-15, 2021, 2021. @inproceedings{servin2021digital,
title = {Digital twins with embedded particle simulation},
author = {Martin Servin and Folke Vesterlund and Erik Wallin},
url = {http://umu.diva-portal.org/smash/record.jsf?language=sv&pid=diva2%3A1499113&dswid=-3497
http://umu.diva-portal.org/smash/get/diva2:1499113/FULLTEXT01.pdf},
year = {2021},
date = {2021-01-01},
booktitle = {14th World Congress in Computational Mechanics (WCCM) ECCOMAS Congress, Virtual, January 11-15, 2021},
keywords = {Algoryx},
pubstate = {published},
tppubtype = {inproceedings}
}
|
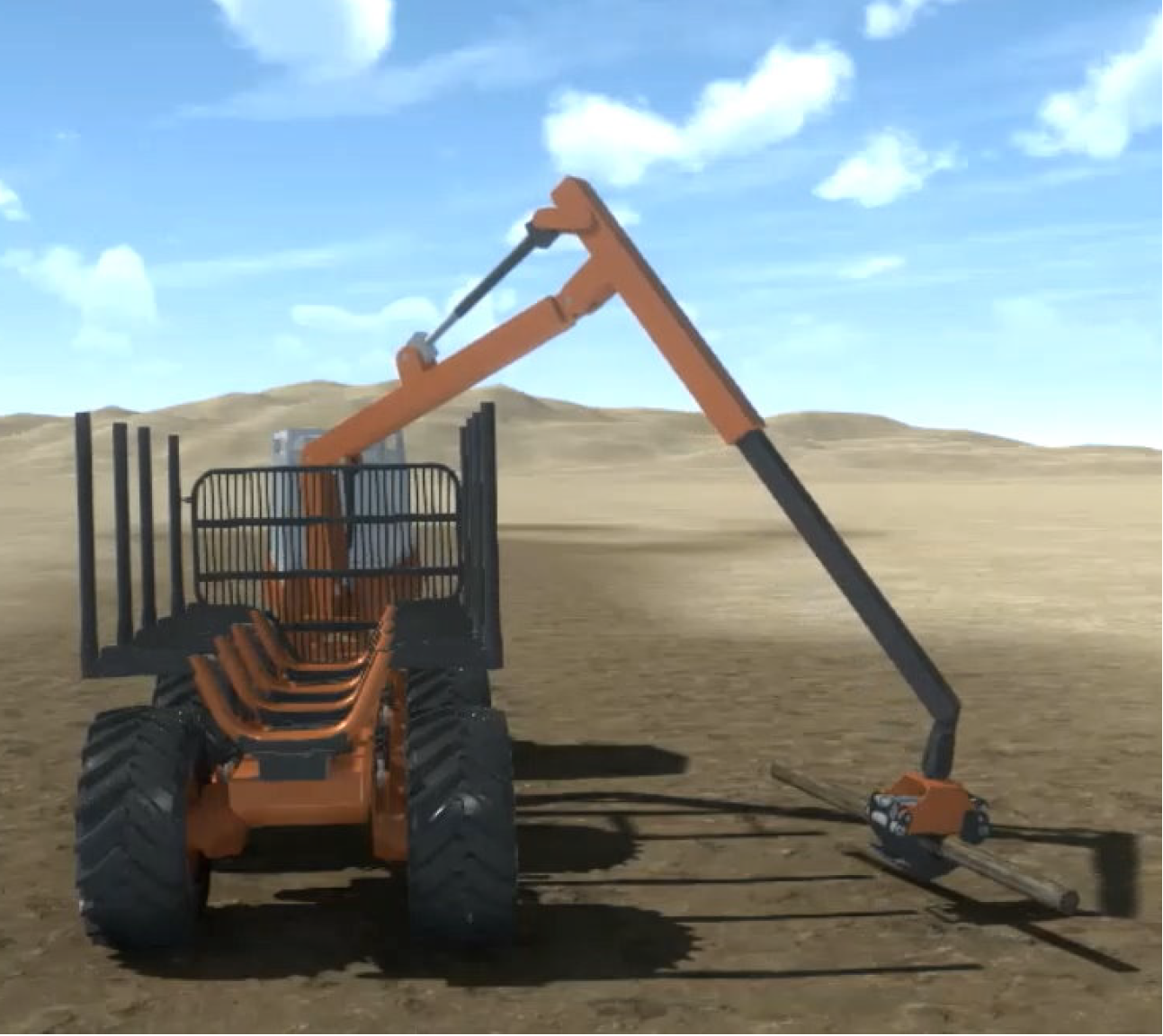 | Andersson, Jennifer; Bodin, Kenneth; Lindmark, Daniel; Servin, Martin; Wallin, Erik: Reinforcement Learning Control of a Forestry Crane Manipulator. In: IEEE/RSJ International Conference on Intelligent Robots and Systems (IROS 2021), Sep. 27-Oct. 1st, 2021, Prague, Czech Republic (2021). arXiv:2103.02315, 2021. @article{andersson2021reinforcement,
title = {Reinforcement Learning Control of a Forestry Crane Manipulator},
author = {Jennifer Andersson and Kenneth Bodin and Daniel Lindmark and Martin Servin and Erik Wallin},
url = {https://arxiv.org/abs/2103.02315
https://arxiv.org/pdf/2103.02315
https://www.algoryx.se/papers/rlc-crane/
https://youtu.be/7xwMlS5uqxs},
year = {2021},
date = {2021-01-01},
journal = {IEEE/RSJ International Conference on Intelligent Robots and Systems (IROS 2021), Sep. 27-Oct. 1st, 2021, Prague, Czech Republic (2021). arXiv:2103.02315},
abstract = {Forestry machines are heavy vehicles performing complex manipulation tasks in unstructured production forest environments. Together with the complex dynamics of the on-board hydraulically actuated cranes, the rough forest terrains have posed a particular challenge in forestry automation. In this study, the feasibility of applying reinforcement learning control to forestry crane manipulators is investigated in a simulated environment. Our results show that it is possible to learn successful actuator-space control policies for energy efficient log grasping by invoking a simple curriculum in a deep reinforcement learning setup. Given the pose of the selected logs, our best control policy reaches a grasping success rate of 97%. Including an energy-optimization goal in the reward function, the energy consumption is significantly reduced compared to control policies learned without incentive for energy optimization, while the increase in cycle time is marginal. The energy-optimization effects can be observed in the overall smoother motion and acceleration profiles during crane manipulation.},
keywords = {Algoryx},
pubstate = {published},
tppubtype = {article}
}
Forestry machines are heavy vehicles performing complex manipulation tasks in unstructured production forest environments. Together with the complex dynamics of the on-board hydraulically actuated cranes, the rough forest terrains have posed a particular challenge in forestry automation. In this study, the feasibility of applying reinforcement learning control to forestry crane manipulators is investigated in a simulated environment. Our results show that it is possible to learn successful actuator-space control policies for energy efficient log grasping by invoking a simple curriculum in a deep reinforcement learning setup. Given the pose of the selected logs, our best control policy reaches a grasping success rate of 97%. Including an energy-optimization goal in the reward function, the energy consumption is significantly reduced compared to control policies learned without incentive for energy optimization, while the increase in cycle time is marginal. The energy-optimization effects can be observed in the overall smoother motion and acceleration profiles during crane manipulation. |
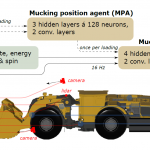 | Backman, Sofi; Lindmark, Daniel; Bodin, Kenneth; Servin, Martin; Mörk, Joakim; Löfgren, Håkan: Continuous control of an underground loader using deep reinforcement learning. In: Machines, vol. 9, no. 10, pp. 216, 2021. @article{backman2021continuous,
title = {Continuous control of an underground loader using deep reinforcement learning},
author = {Sofi Backman and Daniel Lindmark and Kenneth Bodin and Martin Servin and Joakim Mörk and Håkan Löfgren},
url = {https://www.mdpi.com/2075-1702/9/10/216
https://www.mdpi.com/2075-1702/9/10/216/pdf
https://www.algoryx.se/papers/drl-loader/
https://youtu.be/RzDTFZW26H0},
doi = { doi.org/10.3390/machines9100216},
year = {2021},
date = {2021-01-01},
journal = {Machines},
volume = {9},
number = {10},
pages = {216},
abstract = {Reinforcement learning control of an underground loader is investigated in simulated environment, using a multi-agent deep neural network approach. At the start of each loading cycle, one agent selects the dig position from a depth camera image of the pile of fragmented rock. A second agent is responsible for continuous control of the vehicle, with the goal of filling the bucket at the selected loading point, while avoiding collisions, getting stuck, or losing ground traction. It relies on motion and force sensors, as well as on camera and lidar. Using a soft actor-critic algorithm the agents learn policies for efficient bucket filling over many subsequent loading cycles, with clear ability to adapt to the changing environment. The best results, on average 75% of the max capacity, are obtained when including a penalty for energy usage in the reward.},
keywords = {Algoryx},
pubstate = {published},
tppubtype = {article}
}
Reinforcement learning control of an underground loader is investigated in simulated environment, using a multi-agent deep neural network approach. At the start of each loading cycle, one agent selects the dig position from a depth camera image of the pile of fragmented rock. A second agent is responsible for continuous control of the vehicle, with the goal of filling the bucket at the selected loading point, while avoiding collisions, getting stuck, or losing ground traction. It relies on motion and force sensors, as well as on camera and lidar. Using a soft actor-critic algorithm the agents learn policies for efficient bucket filling over many subsequent loading cycles, with clear ability to adapt to the changing environment. The best results, on average 75% of the max capacity, are obtained when including a penalty for energy usage in the reward. |
2020
|
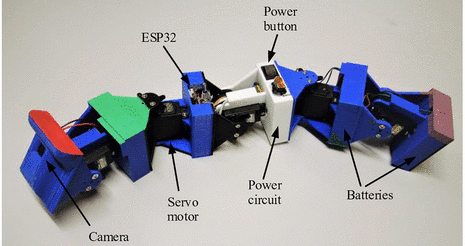 | Li, Guoyuan; Waldum, Håkon Bjerkgaard; Grindvik, Marcus Olai; Jørundl, Ruben Svedal; Zhang, Houxiang: Development of a vision-based target exploration system for snake-like robots in structured environments. In: International Journal of Advanced Robotic Systems, pp. 1-11, 2020. @article{Li2020,
title = {Development of a vision-based target exploration system for snake-like robots in structured environments},
author = {Guoyuan Li and Håkon Bjerkgaard Waldum and Marcus Olai Grindvik and Ruben Svedal Jørundl and Houxiang Zhang},
url = {https://journals.sagepub.com/doi/full/10.1177/1729881420936141
https://www.researchgate.net/publication/342710008_Development_of_a_vision-based_target_exploration_system_for_snake-like_robots_in_structured_environments},
doi = {10.1177/1729881420936141},
year = {2020},
date = {2020-07-06},
journal = {International Journal of Advanced Robotic Systems},
pages = {1-11},
abstract = {Applying snake-like robots to environmental exploration has been a hot topic for years. How to achieve free navigation for target search in a complex environment in a safe and efficient manner is one of the main tasks that researchers in the field of robotics currently face. This article presents a target exploration system that takes advantages of visual sensing to navigate the snake-like robot in structured environments. Two cameras are utilized in the system. The first one is mounted on the head of the snake-like robot for target recognition and the other is an overhead camera which is responsible for locating the robot and identifying surrounding obstacles. All dead ends in the environment can thus be recognized using a template-based method. A search strategy for traversal of the dead ends is employed for generating exploration paths. Several gaits are developed for the snake-like robot. By switching between these gaits, the snake-like robot is able to follow the paths to search for the target. Two experiments are conducted in a maze environment. The experimental results validate the effectiveness of the proposed system for snake-like robots exploring in structured environments.},
keywords = {External},
pubstate = {published},
tppubtype = {article}
}
Applying snake-like robots to environmental exploration has been a hot topic for years. How to achieve free navigation for target search in a complex environment in a safe and efficient manner is one of the main tasks that researchers in the field of robotics currently face. This article presents a target exploration system that takes advantages of visual sensing to navigate the snake-like robot in structured environments. Two cameras are utilized in the system. The first one is mounted on the head of the snake-like robot for target recognition and the other is an overhead camera which is responsible for locating the robot and identifying surrounding obstacles. All dead ends in the environment can thus be recognized using a template-based method. A search strategy for traversal of the dead ends is employed for generating exploration paths. Several gaits are developed for the snake-like robot. By switching between these gaits, the snake-like robot is able to follow the paths to search for the target. Two experiments are conducted in a maze environment. The experimental results validate the effectiveness of the proposed system for snake-like robots exploring in structured environments. |
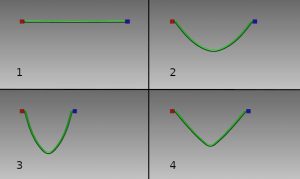 | Laezza, Rita; Karayiannidis, Yiannis: Shape Control of Elastoplastic Deformable Linear Objects through Reinforcement Learning. IEEE/RSJ International Conference on Intelligent Robots and Systems (IROS)
Las Vegas (Virtual), USA, 2020-10-25 - 2020-10-29, 2020. @conference{laezzashape2020,
title = {Shape Control of Elastoplastic Deformable Linear Objects through Reinforcement Learning},
author = {Rita Laezza and Yiannis Karayiannidis},
url = {https://ras.papercept.net/proceedings/IROS20/3496.pdf},
year = {2020},
date = {2020-01-01},
booktitle = {IEEE/RSJ International Conference on Intelligent Robots and Systems (IROS)
Las Vegas (Virtual), USA, 2020-10-25 - 2020-10-29},
abstract = {Deformable object manipulation tasks have longbeen regarded as challenging robotic problems. However, untilrecently, very little work had been done on the subject, withmost robotic manipulation methods being developed for rigidobjects. As machine learning methods are becoming morepowerful, there are new model-free strategies to explore forthese objects, which are notoriously hard to model. This paperfocuses on shape control problems for Deformable Linear Objects (DLOs). Despite being one of the most researched classesof DLOs in terms of geometry, no other paper has focusedon materials with elastoplastic properties. Therefore, a novelshape control task, requiring permanent plastic deformationis implemented in a simulation environment. ReinforcementLearning methods are used to learn a continuous controlpolicy. To that end, a discrete curvature measure is usedas a low-dimensional state representation and as part of anintuitive reward function. Finally, three state-of-the-art actor-critic algorithms are compared on the proposed environmentand successfully achieve the goal shape.},
keywords = {External},
pubstate = {published},
tppubtype = {conference}
}
Deformable object manipulation tasks have longbeen regarded as challenging robotic problems. However, untilrecently, very little work had been done on the subject, withmost robotic manipulation methods being developed for rigidobjects. As machine learning methods are becoming morepowerful, there are new model-free strategies to explore forthese objects, which are notoriously hard to model. This paperfocuses on shape control problems for Deformable Linear Objects (DLOs). Despite being one of the most researched classesof DLOs in terms of geometry, no other paper has focusedon materials with elastoplastic properties. Therefore, a novelshape control task, requiring permanent plastic deformationis implemented in a simulation environment. ReinforcementLearning methods are used to learn a continuous controlpolicy. To that end, a discrete curvature measure is usedas a low-dimensional state representation and as part of anintuitive reward function. Finally, three state-of-the-art actor-critic algorithms are compared on the proposed environmentand successfully achieve the goal shape. |
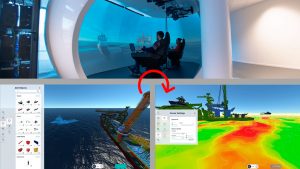 | Major, Pierre; Zhang, Houxiang; Hildre, Hans Petter; Edet, Mathieu: Virtual prototyping of offshore operations: a review. In: Ship Technology Research, pp. 1–18, 2020. @article{major2020virtual,
title = {Virtual prototyping of offshore operations: a review},
author = {Pierre Major and Houxiang Zhang and Hans Petter Hildre and Mathieu Edet},
doi = {10.1080/09377255.2020.1831840},
year = {2020},
date = {2020-01-01},
journal = {Ship Technology Research},
pages = {1--18},
publisher = {Taylor & Francis},
abstract = {Virtual prototyping of offshore operations (VPOO) is performed to plan and validate planning of infrequent or demanding operations characterized by high risk and low margins of error in hostile and remote environments distant from emergency response bases that require expensive equipment. Key elements of VPOO is the rapidity of virtual prototyping and the human-centric approach necessitating high quality visuals and real-time time-domain simulation. This survey reviews publications, commercial software and simulators, and regulations on offshore operations. Findings indicate that the VPOO is not common in the industry, offshore operation regulations lag behind the state of the art in industry in terms of mission planning, and this field has been subject to scarce commercial and scientific scrutiny so far. A discussion of future developments and trends concludes the paper.},
keywords = {External},
pubstate = {published},
tppubtype = {article}
}
Virtual prototyping of offshore operations (VPOO) is performed to plan and validate planning of infrequent or demanding operations characterized by high risk and low margins of error in hostile and remote environments distant from emergency response bases that require expensive equipment. Key elements of VPOO is the rapidity of virtual prototyping and the human-centric approach necessitating high quality visuals and real-time time-domain simulation. This survey reviews publications, commercial software and simulators, and regulations on offshore operations. Findings indicate that the VPOO is not common in the industry, offshore operation regulations lag behind the state of the art in industry in terms of mission planning, and this field has been subject to scarce commercial and scientific scrutiny so far. A discussion of future developments and trends concludes the paper. |